
X . I
Definition Image Processing (Image Processing)
The image processing is any form of signal processing where the input is an image, such as a photo or video frame, while the output of the image processing can be either an image or a number of characteristics or parameters associated with the image. Most image-processing techniques involve or treat the image as a two-dimensional signal and applying standard signal-processing techniques to it, usually it refers to the processing of digital images, but can also be used for optical and analog image processing. The acquisition of the image or with the image input in the first place is referred to as imaging.
An image processing and image analysis processing that involve visual perception. This process has the characteristics of data input and output information in the form of images. The term digital image processing is generally defined as a two-dimensional image processing by a computer. In a broader definition, digital image processing also includes all two-dimensional data. The digital image is a line of real or complex numbers are represented by specific bits.
Generally, digital image, rectangular or square (on some existing imaging systems are shaped hexagons) that has a certain width and height. This is usually expressed in the number of dots, or pixels, so the image size is always worth a round. Each point has a corresponding coordinate position in the image. These coordinates are usually expressed in positive integers, which can be started from 0 or 1 depending on the system used. Each point also has a numeric value that represents the digital information is represented by the point.
The format of the digital image data is closely related to color. In most cases, primarily for visual appearance, the value of digital data representing the colors of the image are processed. Digital image format that is widely used is a binary image (monochrome), Grayscale image (gray scale), Image Color (true color), and indexed color image.
The device image processing system are grouped into three parts:1. Hardware
2. The software
3. Human Intelligence (brain ware)
The third grouping of the image processing system has become essential in image processing. Where the computers are now almost said to meet the standard specifications to perform image processing. But in reality there are many other devices that need to be completed to perform image processing, not just computers, but other devices that do not include a computer or a PC.
X . II
Image Processing Operations
1.Repair: the quality of the image (image enhacement)Objective: improve the quality of the image by manipulating the parameters of the image.
Image enhancement operations:• Improved contrast dark / bright• Improved edges of the objects (edge enhancement)• Refine (sharpening)• Giving false color (pseudocoloring)• Screening noise (noise filtering)
2.Pemugaran image (image restoration)Goal: to eliminate defects in citra.Perbedaannya with image enhancement: an unknown cause image degradation.
Image restoration operations:• Removal of ambiguities (deblurring)• The elimination of noise (noise)
3.Pemampatan image (image compression)Objective: the image is represented in a more compact form, making the need less memory, but while maintaining the image quality (eg, on BMP into JPG)
4.Segmentasi image (image segmentation)Objective: break an image into multiple segments with a certain criterion.Closely related to pattern recognition.
5.Pengorakan image (image analysis)Objective: calculating the amount of the image to produce a quantitative description. Necessary to localize the desired object from its surroundings
Operation image:-Pendeteksian Object edges (edge detection)-Ekstraksi Limit (boundary)-Represenasi Area (region)
6.Rekonstruksi image (Image recontruction)Objective: to reshape the image of the object of some projections.
• Application of Image Processing and Pattern Recognition
• Trade Sector-Pembacaan Bar code at goods in supermarkets-Introduction Letters / numbers on the form automatically• Military Sector-Identify Missile through visual sensors-Mengidentifikasi Type of enemy planes• Field of MedicineCancer-detection by X-rays-Rekonstruksi Fetal sonogram photo• Field Biology-Penenalan Chromosome through microscopic image•Data communication-Pemampatan Image transmission•EntertainmentMPEG video - encriptyon
• Robotics-Visual Guided autonomous navigation•Mapping-Klasifikasi Ground through the use of aerial photographs•Geology-Identify Types of rocks through aerial photos•Law-Introduction Fingerprints-Introduction Inmate photo
Image Processing Functions (Image Processing)
Image processing has several functions, including:-For The process of improving the quality of the image to be easily interpreted by humans or computers-used To image processing techniques to transform the image into another imageFor example: compression of the image (image compression)-As The initial process (preprocessing) of computer vision.
X . III
Device Image Processing System
1. Hardware (Hardware)
Hardware: the form of the computer and the instrument (supporting device). Data contained in Image Processing System is processed through the hardware. Hardware in the image processing are divided into three groups:
• Tools inputs (input) as a means to enter data into a computer network. Example: scanner, digitizer, CD-ROM, Pendrive.
• processing equipment, a computer system that functions to process, analyze and store incoming data as needed, for example: CPU, tape drives, disk drives.
• Tool output (output) that serve geographic information to serve as the data in the GIS process, for example: VDU, plotter, printer
X . IIII

Digital imaging
Digital Imaging (UK) or Digital Imaging is the creation of digital images, usually of a physical scene. The term is often taken to imply or include the processing, compression, storage, printing, and display the image. The most common method is to digital photography with digital cameras, but other methods are also used.
History
Digital
Imaging developed in the 1960s and 1970s, mostly to avoid operational
weaknesses film cameras, for scientific and military missions including
KH-11 program. As digital technology becomes cheaper in the next few decades, replaced the old movies for a variety of purposes. The digital image was first produced in 1920, by drawing Bartlane cable transmission system. British inventor, Harry G. Bartholomew and Maynard D. McFarlane, developed this method. The
process consists of "a series of negatives on zinc plates are open for
long periods of time, resulting in a different density,". Bartlane cable transmission system image produced on both the
transmitter and receiver end to suppress data card or tape that was
created as an image.
In 1957, Russell A. Kirsch generating devices produced digital data that can be stored in a computer; The use of drum scanners and photomultiplier tubes. In
the early 1960s, when it developed a compact, lightweight, portable
equipment for non destructive testing onboard naval aircraft, Frederick
G. Weighart and James F. McNulty in the Industrial Automation,
Inc., later, in El Segundo ,
California found the first apparatus that produces digital images in
real-time, which is a digital radiographic fluoroscopic images. Square-wave signals that are detected by the pixel of the cathode ray tube to create an image.
These ideas different scanning is the basis of the design of the first digital cameras. Early camera took a long time to capture the image and poorly suited for consumer purposes. Not until the development of CCD (charge-coupled device) that digital cameras really took off. CCD
became part of the imaging system used in the telescope, the first
black and white digital camera and camcorder in the 1980s. Finally added to the color CCD camera and a regular feature today.
Changes in the environment
Great strides have been made in the field of digital imaging. Negative and exposure is a foreign concept to many people, and the
first digital image in 1920 eventually led to the equipment cheaper, yet
simple software is getting stronger, and the growth of the Internet.
Constant
progress and production of physical equipment and hardware associated
with digital imaging environment has been carried around the field. Of the camera and webcam for the printer and scanner, the hardware becomes leaner, thinner, faster, and cheaper. As the cost of equipment decreases, the market for new fans widen,
allowing more consumers to experience the thrill of creating their own
images.
Everyday personal laptop, desktop family, and computer companies are capable of handling photography software. Our
computer is a more powerful engine with increased capacity for running
software programs-especially the kind of digital imaging. And software quickly become both smart and simple. Although the function of the program currently reaches levels
appropriate editing and even 3D rendering, the user interface is
designed to be friendly to the advanced users as well as a fan first.
Internet
allows to edit, view and share digital photos and graphics A quick
browse around the web can easily arise works of graphic art from the
artists, news photos from around the world, drawing the company's
products and services, and more. The Internet has clearly proven itself a catalyst in stimulating the growth of digital imaging.
Sharing photos online changed the way we understand photography and photographers. Online
sites such as Flickr, Shutterfly, and Instagram provides billions
ability to share their photography, whether they are amateurs or
professionals. Photography has changed from a luxury media communication and shared more than a moment in time. The subject also has changed. The images used to mainly taken from people and families. Now, we take them out of anything. We can document the day and share it with everyone with the touch of our fingers.
Progresstheory Applications
Although
the theory quickly became a reality in society today's technology, the
possibilities for digital imaging are wide open. One of the main applications are still in the works is that the child's safety and protection. How can we use digital imaging to protect our children? Kodak program, Kids Digital Identification Software (KIDS) can answer that question. Beginning
including digital imaging kit that will be used to develop student
identification photograph, which will be useful as medical emergencies
and crimes. Version is more powerful and advanced applications such as this are
still growing, with an improved feature is constantly tested and
written.
But parents and schools are not the only people who see benefits in a database like this. Office
criminal investigations, such as the area around the police, the state
crime lab, and even federal agencies realize the importance of digital
imaging in analyzing fingerprints and evidence, make arrests, and
maintain a safe community. As the field of digital imaging to be raised, so does our ability to protect the public.
Digital
imaging can be closely linked with the theory of social presence,
especially when referring to the social media aspect of the photos taken
by our phones. There
are many different definitions of the theory of social presence, but
the two that clearly define what it will be "the extent to which people
are considered as real" (Gunawardena, 1995), and "the ability to project
themselves socially and emotionally as a real person" (Garrison, 2000). Digital
imaging allows one to realize their social life through the images in
order to give a sense of their presence into cyberspace. The
presence of the images act as an extension of oneself to others,
provide a digital representation of what they do and who they are with. Digital
imaging within the meaning of the camera on the phone to help
facilitate this effect with the presence of friends in social media. Alexander
(2012) states, "the presence and representation of the image etched in
our reflection ... This, of course, the presence of modified ... no
confusing picture with a representation of reality. But we allow
ourselves to be taken in by those representations, and only that
'representation' was able to demonstrate the activity which is not
present in a believable way. "Therefore, digital imaging allow ourselves
to be represented in a way that reflects our social existence.
Method
A digital photograph can be created directly from the physical scene with a camera or similar device. Alternatively,
digital images can be obtained from other images in analog media, such
as photographs, photographic film or paper printed, the image scanner or
similar device. Many
images-such as technical obtained by tomography equipment, side-scan
sonar, or radio-telescope actually complex processing of data obtained
by the non-image. Weather radar map as seen on the television news is a typical example. Digitizing analog real world data is known as digitization, and involves sampling (discretization) and quantitation.
Finally, digital images can also be calculated from the geometric model or mathematical formulas. In this case the image synthesis more appropriate name, and more commonly known as rendering.
Authentication
of digital images is a problem for the providers and manufacturers
of digital images such as health care organizations, law enforcement
agencies and insurance companies. There are several methods that appear in forensic photography to
analyze digital images and determine whether it has been altered.
Previous
digital imaging depends on chemical and mechanical processes, now all
these processes have been converted to electronic. Some
things need to be done for digital imaging occurs, the light energy is
converted into electrical energy to think of the grid with millions of
tiny solar cells. Each specific conditions generate electric charge. The fee for each "solar cells" transportable and communicated to the firmware to be interpreted. Firmware is what you understand and translate the color and quality of the other light. Pixel is what you look forward, with varying intensities they create and cause a different color, creating images or pictures. Finally firmware records the information for future and further reproduction.profit
There are several benefits of digital imaging. First, the process allows easy access photos and word documents. Google is at the forefront of this 'revolution' with a mission to digitize the world's books. Digitisation will make books searchable, thereby making the
participating libraries, such as Stanford University and the University
of California Berkley, accessible worldwide. LossDigital imaging Critics cite some negative consequences. The
increase in "flexibility in obtaining better quality images for the
reader" will tempt editors, photographers and journalists to manipulate
photos. In
addition, the "staff photographers will no longer be a photojournalist,
but the camera operator ... as the editor has the power to decide what
they want 'photographed'". Legal
constraints, including copyright, raises other concerns: copyright
infringement will occur as digitized documents and copying becomes
easier.
X . IIIII
Controlling image processing: providing extensible, run-time configurable functionality on autonomous robos
The dynamic nature of autonomous robos ' tasks requires that their image
processing operations are tightly coupled to those actions within their
control systems which require the visual information. While there are
many image processing libraries that provide the raw image processing
functionality required for autonomous robo applications, these
libraries do not provide the additional functionality necessary for
transparently binding image processing operations within a robos
control system. In particular such libraries lack facilities for process
scheduling, sequencing, concurrent execution and resource management.
The paper describes the design and implementation of an enabling
extensible system-RECIPE-for providing image processing functionality in
a form that is convenient for robo control together with concrete
implementation examples.
X . IIIIII
Computer Vision
Computer vision is an interdisciplinary field that deals with how computers can be made to gain high-level understanding from digital images or videos. From the perspective of engineering, it seeks to automate tasks that the human visual system can do.
Computer vision tasks include methods for acquiring, processing, analyzing and understanding digital images, and in general, deal with the extraction of high-dimensional data from the real world in order to produce numerical or symbolic information, e.g., in the forms of decisions. Understanding in this context means the transformation of visual images (the input of the retina) into descriptions of the world that can interface with other thought processes and elicit appropriate action. This image understanding can be seen as the disentangling of symbolic information from image data using models constructed with the aid of geometry, physics, statistics, and learning theory.
As a scientific discipline, computer vision is concerned with the theory behind artificial systems that extract information from images. The image data can take many forms, such as video sequences, views from multiple cameras, or multi-dimensional data from a medical scanner. As a technological discipline, computer vision seeks to apply its theories and models for the construction of computer vision systems.
Sub-domains of computer vision include scene reconstruction, event detection, video tracking, object recognition, object pose estimation, learning, indexing, motion estimation, and image restoration.
History
In the late 1960s, computer vision began at universities that were pioneering artificial intelligence. It was meant to mimic the human visual system, as a stepping stone to endowing robots with intelligent behavior. In 1966, it was believed that this could be achieved through a summer project, by attaching a camera to a computer and having it "describe what it saw".What distinguished computer vision from the prevalent field of digital image processing at that time was a desire to extract three-dimensional structure from images with the goal of achieving full scene understanding. Studies in the 1970s formed the early foundations for many of the computer vision algorithms that exist today, including extraction of edges from images, labeling of lines, non-polyhedral and polyhedral modeling, representation of objects as interconnections of smaller structures, optical flow, and motion estimation.
The next decade saw studies based on more rigorous mathematical analysis and quantitative aspects of computer vision. These include the concept of scale-space, the inference of shape from various cues such as shading, texture and focus, and contour models known as snakes. Researchers also realized that many of these mathematical concepts could be treated within the same optimization framework as regularization and Markov random fields. By the 1990s, some of the previous research topics became more active than the others. Research in projective 3-D reconstructions led to better understanding of camera calibration. With the advent of optimization methods for camera calibration, it was realized that a lot of the ideas were already explored in bundle adjustment theory from the field of photogrammetry. This led to methods for sparse 3-D reconstructions of scenes from multiple images. Progress was made on the dense stereo correspondence problem and further multi-view stereo techniques. At the same time, variations of graph cut were used to solve image segmentation. This decade also marked the first time statistical learning techniques were used in practice to recognize faces in images (see Eigenface). Toward the end of the 1990s, a significant change came about with the increased interaction between the fields of computer graphics and computer vision. This included image-based rendering, image morphing, view interpolation, panoramic image stitching and early light-field rendering.
Recent work has seen the resurgence of feature-based methods, used in conjunction with machine learning techniques and complex optimization frameworks.
hub to hub relation
Areas of artificial intelligence deal with autonomous planning or deliberation for robotical systems to navigate through an environment. A detailed understanding of these environments is required to navigate through them. Information about the environment could be provided by a computer vision system, acting as a vision sensor and providing high-level information about the environment and the robot.Artificial intelligence and computer vision share other topics such as pattern recognition and learning techniques. Consequently, computer vision is sometimes seen as a part of the artificial intelligence field or the computer science field in general.
Solid-state physics is another field that is closely related to computer vision. Most computer vision systems rely on image sensors, which detect electromagnetic radiation, which is typically in the form of either visible or infra-red light. The sensors are designed using quantum physics. The process by which light interacts with surfaces is explained using physics. Physics explains the behavior of optics which are a core part of most imaging systems. Sophisticated image sensors even require quantum mechanics to provide a complete understanding of the image formation process. Also, various measurement problems in physics can be addressed using computer vision, for example motion in fluids.
A third field which plays an important role is neurobiology, specifically the study of the biological vision system. Over the last century, there has been an extensive study of eyes, neurons, and the brain structures devoted to processing of visual stimuli in both humans and various animals. This has led to a coarse, yet complicated, description of how "real" vision systems operate in order to solve certain vision related tasks. These results have led to a subfield within computer vision where artificial systems are designed to mimic the processing and behavior of biological systems, at different levels of complexity. Also, some of the learning-based methods developed within computer vision (e.g. neural net and deep learning based image and feature analysis and classification) have their background in biology.
Some strands of computer vision research are closely related to the study of biological vision – indeed, just as many strands of AI research are closely tied with research into human consciousness, and the use of stored knowledge to interpret, integrate and utilize visual information. The field of biological vision studies and models the physiological processes behind visual perception in humans and other animals. Computer vision, on the other hand, studies and describes the processes implemented in software and hardware behind artificial vision systems. Interdisciplinary exchange between biological and computer vision has proven fruitful for both fields.
Yet another field related to computer vision is signal processing. Many methods for processing of one-variable signals, typically temporal signals, can be extended in a natural way to processing of two-variable signals or multi-variable signals in computer vision. However, because of the specific nature of images there are many methods developed within computer vision which have no counterpart in processing of one-variable signals. Together with the multi-dimensionality of the signal, this defines a subfield in signal processing as a part of computer vision.
Beside the above-mentioned views on computer vision, many of the related research topics can also be studied from a purely mathematical point of view. For example, many methods in computer vision are based on statistics, optimization or geometry. Finally, a significant part of the field is devoted to the implementation aspect of computer vision; how existing methods can be realized in various combinations of software and hardware, or how these methods can be modified in order to gain processing speed without losing too much performance.
The fields most closely related to computer vision are image processing, image analysis and machine vision. There is a significant overlap in the range of techniques and applications that these covers. This implies that the basic techniques that are used and developed in these fields are more or less identical, something which can be interpreted as there is only one field with different names. On the other hand, it appears to be necessary for research groups, scientific journals, conferences and companies to present or market themselves as belonging specifically to one of these fields and, hence, various characterizations which distinguish each of the fields from the others have been presented.
Computer vision is, in some ways, the inverse of computer graphics. While computer graphics produces image data from 3D models, computer vision often produces 3D models from image data. There is also a trend towards a combination of the two disciplines, e.g., as explored in augmented reality.
The following characterizations appear relevant but should not be taken as universally accepted:
- Image processing and image analysis tend to focus on 2D images, how to transform one image to another, e.g., by pixel-wise operations such as contrast enhancement, local operations such as edge extraction or noise removal, or geometrical transformations such as rotating the image. This characterization implies that image processing/analysis neither require assumptions nor produce interpretations about the image content.
- Computer vision includes 3D analysis from 2D images. This analyzes the 3D scene projected onto one or several images, e.g., how to reconstruct structure or other information about the 3D scene from one or several images. Computer vision often relies on more or less complex assumptions about the scene depicted in an image.
- Machine vision is the process of applying a range of technologies & methods to provide imaging-based automatic inspection, process control and robot guidance in industrial applications. Machine vision tends to focus on applications, mainly in manufacturing, e.g., vision based autonomous robots and systems for vision based inspection or measurement. This implies that image sensor technologies and control theory often are integrated with the processing of image data to control a robot and that real-time processing is emphasised by means of efficient implementations in hardware and software. It also implies that the external conditions such as lighting can be and are often more controlled in machine vision than they are in general computer vision, which can enable the use of different algorithms.
- There is also a field called imaging which primarily focus on the process of producing images, but sometimes also deals with processing and analysis of images. For example, medical imaging includes substantial work on the analysis of image data in medical applications.
- Finally, pattern recognition is a field which uses various methods to extract information from signals in general, mainly based on statistical approaches and artificial neural networks. A significant part of this field is devoted to applying these methods to image data.
Applications
Applications range from tasks such as industrial machine vision systems which, say, inspect bottles speeding by on a production line, to research into artificial intelligence and computers or robots that can comprehend the world around them. The computer vision and machine vision fields have significant overlap. Computer vision covers the core technology of automated image analysis which is used in many fields. Machine vision usually refers to a process of combining automated image analysis with other methods and technologies to provide automated inspection and robot guidance in industrial applications. In many computer vision applications, the computers are pre-programmed to solve a particular task, but methods based on learning are now becoming increasingly common. Examples of applications of computer vision include systems for:- Automatic inspection, e.g., in manufacturing applications;
- Assisting humans in identification tasks, e.g., a species identification system;
- Controlling processes, e.g., an industrial robot;
- Detecting events, e.g., for visual surveillance or people counting;
- Interaction, e.g., as the input to a device for computer-human interaction;
- Modeling objects or environments, e.g., medical image analysis or topographical modeling;
- Navigation, e.g., by an autonomous vehicle or mobile robot; and
- Organizing information, e.g., for indexing databases of images and image sequences.
One of the most prominent application fields is medical computer vision or medical image processing. This area is characterized by the extraction of information from image data for the purpose of making a medical diagnosis of a patient. Generally, image data is in the form of microscopy images, X-ray images, angiography images, ultrasonic images, and tomography images. An example of information which can be extracted from such image data is detection of tumours, arteriosclerosis or other malign changes. It can also be measurements of organ dimensions, blood flow, etc. This application area also supports medical research by providing new information, e.g., about the structure of the brain, or about the quality of medical treatments. Applications of computer vision in the medical area also includes enhancement of images that are interpreted by humans, for example ultrasonic images or X-ray images, to reduce the influence of noise.
A second application area in computer vision is in industry, sometimes called machine vision, where information is extracted for the purpose of supporting a manufacturing process. One example is quality control where details or final products are being automatically inspected in order to find defects. Another example is measurement of position and orientation of details to be picked up by a robot arm. Machine vision is also heavily used in agricultural process to remove undesirable food stuff from bulk material, a process called optical sorting.
Military applications are probably one of the largest areas for computer vision. The obvious examples are detection of enemy soldiers or vehicles and missile guidance. More advanced systems for missile guidance send the missile to an area rather than a specific target, and target selection is made when the missile reaches the area based on locally acquired image data. Modern military concepts, such as "battlefield awareness", imply that various sensors, including image sensors, provide a rich set of information about a combat scene which can be used to support strategic decisions. In this case, automatic processing of the data is used to reduce complexity and to fuse information from multiple sensors to increase reliability.
Artist's Concept of Rover on Mars, an example of an unmanned land-based vehicle. Notice the stereo cameras mounted on top of the Rover.
Other application areas include:
- Support of visual effects creation for cinema and broadcast, e.g., camera tracking (matchmoving).
- Surveillance.
Representational and control requirements
Image-understanding systems (IUS) include three levels of abstraction as follows: Low level includes image primitives such as edges, texture elements, or regions; intermediate level includes boundaries, surfaces and volumes; and high level includes objects, scenes, or events. Many of these requirements are really topics for further research.The representational requirements in the designing of IUS for these levels are: representation of prototypical concepts, concept organization, spatial knowledge, temporal knowledge, scaling, and description by comparison and differentiation.
While inference refers to the process of deriving new, not explicitly represented facts from currently known facts, control refers to the process that selects which of the many inference, search, and matching techniques should be applied at a particular stage of processing. Inference and control requirements for IUS are: search and hypothesis activation, matching and hypothesis testing, generation and use of expectations, change and focus of attention, certainty and strength of belief, inference and goal satisfaction.
Typical tasks
Each of the application areas described above employ a range of computer vision tasks; more or less well-defined measurement problems or processing problems, which can be solved using a variety of methods. Some examples of typical computer vision tasks are presented below.Recognition
The classical problem in computer vision, image processing, and machine vision is that of determining whether or not the image data contains some specific object, feature, or activity. Different varieties of the recognition problem are described in the literature:- Object recognition (also called object classification) – one or several pre-specified or learned objects or object classes can be recognized, usually together with their 2D positions in the image or 3D poses in the scene. Blippar, Google Goggles and LikeThat provide stand-alone programs that illustrate this functionality.
- Identification – an individual instance of an object is recognized. Examples include identification of a specific person's face or fingerprint, identification of handwritten digits, or identification of a specific vehicle.
- Detection – the image data are scanned for a specific condition. Examples include detection of possible abnormal cells or tissues in medical images or detection of a vehicle in an automatic road toll system. Detection based on relatively simple and fast computations is sometimes used for finding smaller regions of interesting image data which can be further analyzed by more computationally demanding techniques to produce a correct interpretation.
Several specialized tasks based on recognition exist, such as:
- Content-based image retrieval – finding all images in a larger set of images which have a specific content. The content can be specified in different ways, for example in terms of similarity relative a target image (give me all images similar to image X), or in terms of high-level search criteria given as text input (give me all images which contains many houses, are taken during winter, and have no cars in them).
Computer vision for people counter purposes in public places, malls, shopping centres
- Pose estimation – estimating the position or orientation of a specific object relative to the camera. An example application for this technique would be assisting a robot arm in retrieving objects from a conveyor belt in an assembly line situation or picking parts from a bin.
- Optical character recognition (OCR) – identifying characters in images of printed or handwritten text, usually with a view to encoding the text in a format more amenable to editing or indexing (e.g. ASCII).
- 2D Code reading Reading of 2D codes such as data matrix and QR codes.
- Facial recognition
- Shape Recognition Technology (SRT) in people counter systems differentiating human beings (head and shoulder patterns) from objects
Motion analysis
Several tasks relate to motion estimation where an image sequence is processed to produce an estimate of the velocity either at each points in the image or in the 3D scene, or even of the camera that produces the images . Examples of such tasks are:- Egomotion – determining the 3D rigid motion (rotation and translation) of the camera from an image sequence produced by the camera.
- Tracking – following the movements of a (usually) smaller set of interest points or objects (e.g., vehicles or humans) in the image sequence.
- Optical flow – to determine, for each point in the image, how that point is moving relative to the image plane, i.e., its apparent motion. This motion is a result both of how the corresponding 3D point is moving in the scene and how the camera is moving relative to the scene.
Scene reconstruction
Given one or (typically) more images of a scene, or a video, scene reconstruction aims at computing a 3D model of the scene. In the simplest case the model can be a set of 3D points. More sophisticated methods produce a complete 3D surface model. The advent of 3D imaging not requiring motion or scanning, and related processing algorithms is enabling rapid advances in this field. Grid-based 3D sensing can be used to acquire 3D images from multiple angles. Algorithms are now available to stitch multiple 3D images together into point clouds and 3D models.Image restoration
The aim of image restoration is the removal of noise (sensor noise, motion blur, etc.) from images. The simplest possible approach for noise removal is various types of filters such as low-pass filters or median filters. More sophisticated methods assume a model of how the local image structures look like, a model which distinguishes them from the noise. By first analysing the image data in terms of the local image structures, such as lines or edges, and then controlling the filtering based on local information from the analysis step, a better level of noise removal is usually obtained compared to the simpler approaches.An example in this field is inpainting.
System methods
The organization of a computer vision system is highly application dependent. Some systems are stand-alone applications which solve a specific measurement or detection problem, while others constitute a sub-system of a larger design which, for example, also contains sub-systems for control of mechanical actuators, planning, information databases, man-machine interfaces, etc. The specific implementation of a computer vision system also depends on if its functionality is pre-specified or if some part of it can be learned or modified during operation. Many functions are unique to the application. There are, however, typical functions which are found in many computer vision systems.- Image acquisition – A digital image is produced by one or several image sensors, which, besides various types of light-sensitive cameras, include range sensors, tomography devices, radar, ultra-sonic cameras, etc. Depending on the type of sensor, the resulting image data is an ordinary 2D image, a 3D volume, or an image sequence. The pixel values typically correspond to light intensity in one or several spectral bands (gray images or colour images), but can also be related to various physical measures, such as depth, absorption or reflectance of sonic or electromagnetic waves, or nuclear magnetic resonance.
- Pre-processing – Before a computer vision method can be
applied to image data in order to extract some specific piece of
information, it is usually necessary to process the data in order to
assure that it satisfies certain assumptions implied by the method.
Examples are
- Re-sampling in order to assure that the image coordinate system is correct.
- Noise reduction in order to assure that sensor noise does not introduce false information.
- Contrast enhancement to assure that relevant information can be detected.
- Scale space representation to enhance image structures at locally appropriate scales.
- Feature extraction – Image features at various levels of complexity are extracted from the image data.[20] Typical examples of such features are
- Lines, edges and ridges.
- Localized interest points such as corners, blobs or points.
- More complex features may be related to texture, shape or motion.
- Detection/segmentation – At some point in the processing a
decision is made about which image points or regions of the image are
relevant for further processing. Examples are
- Selection of a specific set of interest points
- Segmentation of one or multiple image regions which contain a specific object of interest.
- Segmentation of image into nested scene architecture comprised foreground, object groups, single objects or salient object parts (also referred to as spatial-taxon scene hierarchy)
- High-level processing – At this step the input is typically a
small set of data, for example a set of points or an image region which
is assumed to contain a specific object. The remaining processing deals with, for example:
- Verification that the data satisfy model-based and application specific assumptions.
- Estimation of application specific parameters, such as object pose or object size.
- Image recognition – classifying a detected object into different categories.
- Image registration – comparing and combining two different views of the same object.
- Decision making Making the final decision required for the application, for example:
- Pass/fail on automatic inspection applications
- Match / no-match in recognition applications
- Flag for further human review in medical, military, security and recognition applications
Hardware
There are many kinds of computer vision systems, nevertheless all of them contain these basic elements: a power source, at least one image acquisition device (i.e. camera, ccd, etc.), a processor as well as control and communication cables or some kind of wireless interconnection mechanism. In addition, a practical vision system contains software, as well as a display in order to monitor the system. Vision systems for inner spaces, as most industrial ones, contain an illumination system and may be placed in a controlled environment. Furthermore, a completed system includes many accessories like camera supports, cables and connectors.
While traditional broadcast and consumer video systems operate at a rate of 30 frames per second, advances in digital signal processing and consumer graphics hardware has made high-speed image acquisition, processing, and display possible for real-time systems on the order of hundreds to thousands of frames per second. For applications in robotics, fast, real-time video systems are critically important and often can simplify the processing needed for certain algorithms. When combined with a high-speed projector, fast image acquisition allows 3D measurement and feature tracking to be realised.
As of 2016, vision processing units are emerging as a new class of processor, to complement CPUs and GPUs in this role .
X . IIIIIII
Applications of artificial intelligence
Artificial intelligence has been used in a wide range of fields including medical diagnosis, stock trading, robot control, law, remote sensing, scientific discovery and toys. However, due to the AI effect, many AI applications are not perceived as AI. "A lot of cutting edge AI has filtered into general applications, often without being called AI because once something becomes useful enough and common enough it's not labeled AI anymore," Nick Bostrom, a noted philosopher, reports. "Many thousands of AI applications are deeply embedded in the infrastructure of every industry." In the late 90s and early 21st century, AI technology became widely used as elements of larger systems, but the field is rarely credited for these successes. It continues to develop in numerous fields today including:Computer science
AI researchers have created many tools to solve the most difficult problems in computer science. Many of their inventions have been adopted by mainstream computer science and are no longer considered a part of AI. (See AI effect). According to Russell & Norvig (2003, p. 15), all of the following were originally developed in AI laboratories: time sharing, interactive interpreters, graphical user interfaces and the computer mouse, rapid development environments, the linked list data structure, automatic storage management, symbolic programming, functional programming, dynamic programming and object-oriented programming.
Finance
Financial institutions have long used artificial neural network systems to detect charges or claims outside of the norm, flagging these for human investigation.
Use of AI in banking can be tracked back to 1987 when Security Pacific National Bank in USA set-up a Fraud Prevention Task force to counter the unauthorised use of debit cards. Apps like Kasisito and Moneystream are using AI in financial services
Banks use artificial intelligence systems to organize operations, maintain book-keeping, invest in stocks, and manage properties. For example, Kensho is a computer system that is used to analyze how well portfolios perform and predict changes in the market. AI can react to changes overnight or when business is not taking place. In August 2001, robots beat humans in a simulated financial trading competition.
Also, systems are being developed, like Arria, to translate complex data into simple and personable language.
There are also wallets, like Wallet.AI that monitor an individual's spending habits and provides ways to improve them.
AI has also reduced fraud and crime by monitoring behavioral patterns of users for any changes or anomalies.
Hospitals and medicine
Main article: Artificial intelligence in healthcareArtificial neural networks are used as clinical decision support systems for medical diagnosis, such as in Concept Processing technology in EMR software.
Other tasks in medicine that can potentially be performed by artificial intelligence and are beginning to be developed include:
- Computer-aided interpretation of medical images. Such systems help scan digital images, e.g. from computed tomography, for typical appearances and to highlight conspicuous sections, such as possible diseases. A typical application is the detection of a tumor.
- Heart sound analysis
- Watson project is another use of AI in this field, a Q/A program that suggest for doctor's of cancer patients.
- Companion robots for the care of the elderly
- Mining medical records to provide more useful information
- Design treatment plans
- Assist in repetitive jobs including medication management
- Provide consultations
- Drug creation
Heavy industry
Robots have become common in many industries and are often given jobs that are considered dangerous to humans. Robots have proven effective in jobs that are very repetitive which may lead to mistakes or accidents due to a lapse in concentration and other jobs which humans may find degrading.In 2014, China, Japan, the United States, the Republic of Korea and Germany together amounted to 70% of the total sales volume of robots. In the automotive industry, a sector with particularly high degree of automation, Japan had the highest density of industrial robots in the world: 1,414 per 10,000 employees.
Online and telephone customer service
An automated online assistant providing customer service on a web page.
Currently, major companies are investing in AI to handle difficult customer in the future. Google's most recent development analyzes language and converts speech into text. The platform can identify angry customers through their language and respond appropriately.
Companies have been working on different aspects of customer service to improve this aspect of a company.
Digital Genius, an AI start-up, researches the database of information (from past conversations and frequently asked questions) more efficiently and provide prompts to agents to help them resolve queries more efficiently.
IPSoft is creating technology with emotional intelligence to adapt the customer's interaction. The response is linked to the customer's tone, with the objective of being able to show empathy. Another element IPSoft is developing is the ability to adapt to different tones or languages.
Inbenta’s is focused on developing natural language. In other words, on understanding the meaning behind what someone is asking and not just looking at the words used, using context and natural language processing. One customer service element Ibenta has already achieved is its ability to respond in bulk to email queries.
Transportation
Many companies have been progressing quickly in this field with AI.Fuzzy logic controllers have been developed for automatic gearboxes in automobiles. For example, the 2006 Audi TT, VW Touareg and VW Caravell feature the DSP transmission which utilizes Fuzzy Logic. A number of Å koda variants (Å koda Fabia) also currently include a Fuzzy Logic-based controller.
AI in transportation is expected to provide safe, efficient, and reliable transportation while minimizing the impact on the environment and communities. The major challenge to developing this AI is the fact that transportation systems are inherently complex systems involving a very large number of components and different parties, each having different and often conflicting objectives.
Telecommunications maintenance
Many telecommunications companies make use of heuristic search in the management of their workforces, for example BT Group has deployed heuristic search in a scheduling application that provides the work schedules of 20,000 engineers.Toys and games
The 1990s saw some of the first attempts to mass-produce domestically aimed types of basic Artificial Intelligence for education, or leisure. This prospered greatly with the Digital Revolution, and helped introduce people, especially children, to a life of dealing with various types of Artificial Intelligence, specifically in the form of Tamagotchis and Giga Pets, iPod Touch, the Internet, and the first widely released robot, Furby. A mere year later an improved type of domestic robot was released in the form of Aibo, a robotic dog with intelligent features and autonomy.Companies like Mattel have been creating an assortment of AI-enabled toys for kids as young as age three. Using proprietary AI engines and speech recognition tools, they are able to understand conversations, give intelligent responses and learn quickly.
AI has also been applied to video games, for example video game bots, which are designed to stand in as opponents where humans aren't available or desired.
Music
While the evolution of music has always been affected by technology, artificial intelligence has enabled, through scientific advances, to emulate, at some extent, human-like composition.Among notable early efforts, David Cope created an AI called Emily Howell that managed to become well known in the field of Algorithmic Computer Music. The algorithm behind Emily Howell is registered as a US patent.
Other endeavours, like AIVA (Artificial Intelligence Virtual Artist), focus on composing symphonic music, mainly classical music for film scores. It achieved a world first by becoming the first virtual composer to be recognized by a musical professional association.
Artificial intelligences can even produce music usable in a medical setting, with Melomics’s effort to use computer-generated music for stress and pain relief.
Moreover, initiatives such as Google Magenta, conducted by the Google Brain team, want to find out if an artificial intelligence can be capable of creating compelling art.
At Sony CSL Research Laboratory, their Flow Machines software has created pop songs by learning music styles from a huge database of songs. By analyzing unique combinations of styles and optimizing techniques, it can compose in any style.
Aviation
The use of artificial intelligence in simulators is proving to be very useful for the AOD. Airplane simulators are using artificial intelligence in order to process the data taken from simulated flights. Other than simulated flying, there is also simulated aircraft warfare. The computers are able to come up with the best success scenarios in these situations. The computers can also create strategies based on the placement, size, speed and strength of the forces and counter forces. Pilots may be given assistance in the air during combat by computers. The artificial intelligent programs can sort the information and provide the pilot with the best possible maneuvers, not to mention getting rid of certain maneuvers that would be impossible for a human being to perform. Multiple aircraft are needed to get good approximations for some calculations so computer simulated pilots are used to gather data. These computer simulated pilots are also used to train future air traffic controllers.
The system used by the AOD in order to measure performance was the Interactive Fault Diagnosis and Isolation System, or IFDIS. It is a rule based expert system put together by collecting information from TF-30 documents and the expert advice from mechanics that work on the TF-30. This system was designed to be used for the development of the TF-30 for the RAAF F-111C. The performance system was also used to replace specialized workers. The system allowed the regular workers to communicate with the system and avoid mistakes, miscalculations, or having to speak to one of the specialized workers.
The AOD also uses artificial intelligence in speech recognition software. The air traffic controllers are giving directions to the artificial pilots and the AOD wants to the pilots to respond to the ATC's with simple responses. The programs that incorporate the speech software must be trained, which means they use neural networks. The program used, the Verbex 7000, is still a very early program that has plenty of room for improvement. The improvements are imperative because ATCs use very specific dialog and the software needs to be able to communicate correctly and promptly every time.
The Artificial Intelligence supported Design of Aircraft, or AIDA, is used to help designers in the process of creating conceptual designs of aircraft. This program allows the designers to focus more on the design itself and less on the design process. The software also allows the user to focus less on the software tools. The AIDA uses rule based systems to compute its data. This is a diagram of the arrangement of the AIDA modules. Although simple, the program is proving effective.
In 2003, NASA's Dryden Flight Research Center, and many other companies, created software that could enable a damaged aircraft to continue flight until a safe landing zone can be reached. The software compensates for all the damaged components by relying on the undamaged components. The neural network used in the software proved to be effective and marked a triumph for artificial intelligence.
The Integrated Vehicle Health Management system, also used by NASA, on board an aircraft must process and interpret data taken from the various sensors on the aircraft. The system needs to be able to determine the structural integrity of the aircraft. The system also needs to implement protocols in case of any damage taken the vehicle.
News, publishing and writing
The company Narrative Science makes computer generated news and reports commercially available, including summarizing team sporting events based on statistical data from the game in English. It also creates financial reports and real estate analyses. Similarly, the company Automated Insights generates personalized recaps and previews for Yahoo Sports Fantasy Football. The company is projected to generate one billion stories in 2014, up from 350 million in 2013.Echobox is a software company that helps publishers increase traffic by 'intelligently' posting articles on social media platforms such as Facebook and Twitter. By analysing large amounts of data, it learns how specific audiences respond to different articles at different times of the day. It then chooses the best stories to post and the best times to post them. It uses both historical and real-time data to understand to what has worked well in the past as well as what is currently trending on the web.
Another company, called Yseop, uses artificial intelligence to turn structured data into intelligent comments and recommendations in natural language. Yseop is able to write financial reports, executive summaries, personalized sales or marketing documents and more at a speed of thousands of pages per second and in multiple languages including English, Spanish, French & German.
Boomtrain’s is another example of AI that is designed to learn how to best engage each individual reader with the exact articles — sent through the right channel at the right time — that will be most relevant to the reader. It’s like hiring a personal editor for each individual reader to curate the perfect reading experience.
There is also the possibility that AI will write work in the future. In 2016, a Japanese AI co-wrote a short story and almost won a literary prize.
Other
Various tools of artificial intelligence are also being widely deployed in homeland security, speech and text recognition, data mining, and e-mail spam filtering. Applications are also being developed for gesture recognition (understanding of sign language by machines), individual voice recognition, global voice recognition (from a variety of people in a noisy room), facial expression recognition for interpretation of emotion and non verbal cues. Other applications are robot navigation, obstacle avoidance, and object recognition.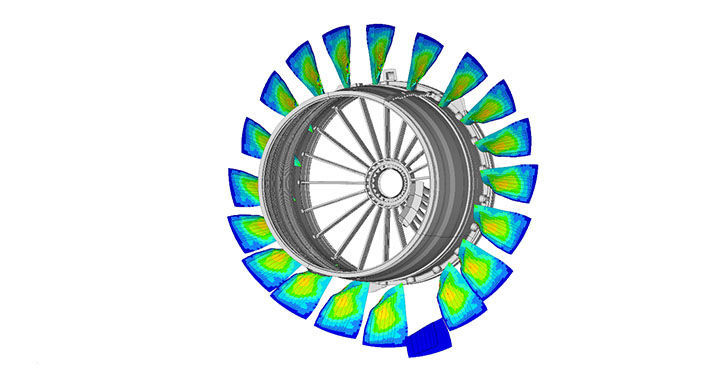
