Robotics Technology - Mobility
Industrial robots are rarely mobile.
Work is generally brought to the robot. A few industrial robots are
mounted on tracks and are mobile within their work

station. Service robots are virtually the only kind of robots that
travel autonomously. Research on robot mobility is extensive. The goal
of the research is usually to have the robot navigate in unstructured
environments while encountering unforeseen obstacles. Some projects
raise the technical barriers by insisting that the locomotion involve
walking, either on two appendages, like humans, or on many, like
insects. Most projects, however, use wheels or tractor mechanisms.
Many kinds of effectors and actuators can be used to move a robot
around. Some categories are:
Wheels are the locomotion effector of choice. Wheeled robots (as well as almost all wheeled
mechanical devices, such as cars) are built to be statically stable.

It is important to remember that wheels can be constructed with as
much variety and innovative flair as legs: wheels can vary in size and
shape, can consist of simple tires, or complex tire patterns, or
tracks, or wheels within cylinders within other wheels spinning in
different directions to provide different types of locomotion
properties. So wheels need not be simple, but typically they are,
because even simple wheels are quite efficient. Having wheels does
not imply holonomicity. 2 or 4-wheeled robots are not usually
holonomic. A popular and efficient design involves 2 differentially-steerable
wheels and a passive caster. Differential steering means that the two
(or more) wheels can be steered separately (individually) and thus
differently. If one wheel can turn in one direction and the other in
the opposite direction, the robot can spin in place. This is very
helpful for following arbitrary trajectories. Tracks are often used
(e.g., tanks).
While most animals use legs to
get around, legged locomotion is a very difficult robotic problem,
especially when compared to wheeled locomotion. First, any robot
needs to be stable (i.e., not wobble and fall over easily). There are
two kinds of stability: static and dynamic. A statically stable
robot can stand still without falling over. This is a useful feature,
but a difficult one to achieve: it requires that there be enough
legs/wheels on the robot to provide sufficient static points of
support. For example, people are not statically stable. In
order to stand up, which appears effortless to us, we are actually
using active control of our balance, though nerves and muscles and
tendons. This balancing is largely unconscious, but must be learned,
so that's why it takes babies a while to get it right, and certain
injuries can make it difficult or impossible. With more legs, static
stability becomes quite simple. In order to remain stable, the
robot's center of gravity (COG) must fall under its polygon of support.
This polygon is basically the projection between all of its support
points onto the surface. So in a two-legged robot, the polygon is
really a line, and the COG cannot be stably aligned with a point on
that line to keep the robot upright. However, a three-legged robot,
with its legs in a tripod organization, and its body above, produces a
stable polygon of support, and is thus statically stable. But what
happens when a statically stable robot lifts a leg and tries to move.
Does its COG stay within the polygon of support? It may or may not,
depending on the geometry. For certain robot geometries, it is
possible (with various numbers of legs) to always stay statically
stable while walking. This is very safe, but it is also very slow and
energy inefficient. A basic assumption of the static gait (statically
stable gait) is that the weight of a leg is negligible compared to
that of the body, so that the total center of gravity (COG) of the
robot is not affected by the leg swing. Based on this assumption, the
conventional static gait is designed so as to maintain the COG of the
robot inside of the support polygon, which is outlined by each support
leg's tip position. The alternative to static stability is
dynamic stability which allows a robot (or animal) to be stable
while moving. For example, one-legged hopping robots are dynamically
stable: they can hop in place or to various destinations, and not fall
over. But they cannot stop and stay standing (this is an inverse
pendulum balancing problem).
A statically stable robot can use
dynamically-stable walking patterns, to be fast, or it can use
statically stable walking. A simple way to think about this is by how
many legs are up in the air during the robot's movement (i.e., gait).
6 legs is the most popular number as they allow for a very stable
walking gait, the tripod gait . If the same three legs move at a time,
this is called the alternating tripod gait. if the legs vary, it is
called the ripple gait. A rectangular 6-legged robot can lift three
legs at a time to move forward, and still retain static stability. How
does it do that? It uses the so-called alternating tripod gait,
a biologically common walking pattern for 6 or more legs. In this
gait, one middle leg on one side and two non-adjacent legs on the
other side of the body lift and move forward at the same time, while
the other 3 legs remain on the ground and keep the robot statically
stable. Roaches move this way, and can do so very quickly. Insects
with more than 6 legs (e.g., centipedes and millipedes), use the
ripple gate. However, when they run really fast, they switch gates to
actually become airborne (and thus not statically stable) for brief
periods of time.
Statically stable walking is
very energy inefficient. As an alternative, dynamic stability enables
a robot to stay up while moving. This requires active control (i.e.,
the inverse pendulum problem). Dynamic stability can allow for greater
speed, but requires harder control. Balance and stability are very
difficult problems in control and robotics, so that is why when you
look at most existing robots, they will have wheels or plenty of legs
(at least 6). Research robotics, of course, is studying single-legged,
two legged, and other dynamically-stable robots, for various
scientific and applied reasons. Wheels are more efficient than legs.
They also do appear in nature, in certain bacteria, so the common myth
that biology cannot make wheels is not well founded. However,
evolution favors lateral symmetry and legs are much easier to evolve,
as is abundantly obvious. However, if you look at population sizes,
insects are most populous animals, and they all have many more than 2
legs.
In solving problems, the Spider
is aided by the spring quality of its 1 mm steel wire legs. Hold one
of its feet in place relative to the body and the mechanism keeps
turning, the obstructed motor consuming less than 40 mA while it bends
the leg. Let go and the leg springs back into shape. As I write this,
the Spider is scrambling up and over my keyboard. Some of its feet get
temporarily stuck between keys, springing loose again as others push
down. It has no trouble whatsoever with this obstacle, nor with any of
the others on my cluttered desk - even though it is still utterly
brainless.
As the feet rise to a maximum of 2 cm
off the floor, a cassette box is about the tallest vertical obstacle
that the Spider is able to step onto. Another limitation is slope.
When asked to sustain a climb angle of more than about 20 degrees, the
Spider rolls over backwards. And even this fairly modest angle
(extremely steep for a car, by the way) requires careful gait control,
making sure that

both
rear legs do not lift at the same time. Improvements are certainly
possible. Increasing step size would require a longer body (more
distance between the legs) and thus a different gear train. A better
choice might be more legs, like 10 or 12 on a longer body, but with
the same size gear wheels. That would give better traction and
climbing ability. And if a third motor is allowed, one might construct
a horizontal hinge in the `backbone'. Make a gear shaft the center of
a nice, tight hinge joint. Then the drive train will function as
before. Using the third motor and a suitable mechanism, the robot
could raise its front part to step onto a tall obstacle, somewhat like
a caterpillar. But turning on the spot becomes difficult.
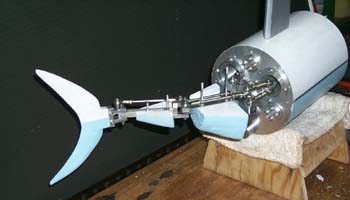 |
Most robots do not
fly or swim. Recently, researchers have been exploring the
possibilities and problems involved with flying and swimming
robots.
Lookahead Navigation for High-Speed Mobile Robots
Recent developments in both defense and commercial sectors have inspired a growing
interest in mobile robot navigation technologies. As look-ahead sensing capabilities
improve, mobile robots will be able to operate at higher speeds and in more varied
environments. This research aims to develop novel planning and control approaches
to meet the challenge.
Operation at high speeds requires the anticipation of obstacles and
terrain changes. In addition, dynamic effects such as friction
saturation and loss of ground contact limit the class of feasible
vehicle trajectories. A lookahead naviation system must be capable of
planning a feasible trajectory through the sensed environment and
controlling the vehicle along that trajectory while remaining robust to
terrain changes and dynamic disturbances.
To achieve this kind of forward-looking, versatile control, we are leveraging the
prediction and constraint-handling capabilities of model predictive control. Model
Predictive Control (MPC) is a flexible, model-based control approach that seeks to
minimize an objective function by optimizing a projected set of control inputs over
a progressive and forward-looking time horizon. Its ability to explicitly consider
constraints, track references, include environmental disturbances, and incorporate
multiple actuation methods make it particularly well-suited for the mobile robot
navigation problem.
|