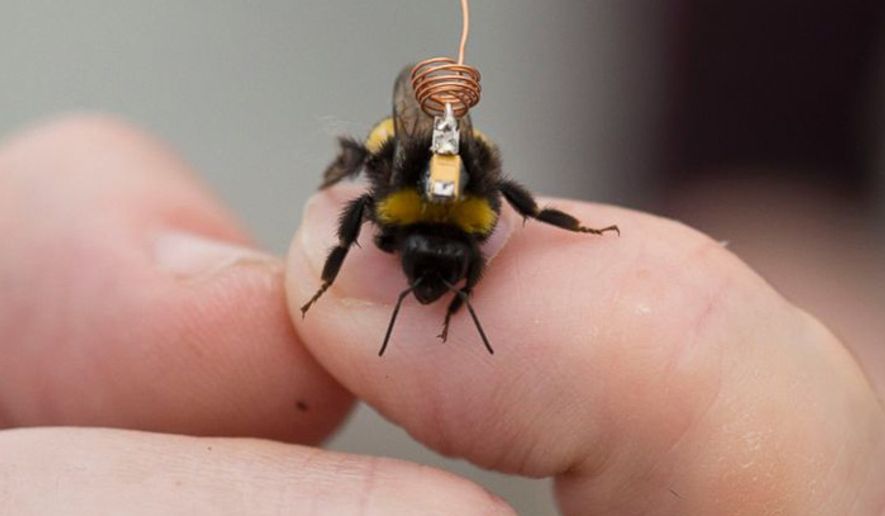
Energy harvesting
Energy harvesting (also known as power harvesting or energy scavenging or ambient power) is the process by which energy is derived from external sources (e.g., solar power, thermal energy, wind energy, salinity gradients, and kinetic energy, also known as ambient energy), captured, and stored for small, wireless autonomous devices, like those used in wearable electronics and wireless sensor networks.
Energy harvesters provide a very small amount of power for low-energy electronics. While the input fuel to some large-scale generation costs resources (oil, coal, etc.), the energy source for energy harvesters is present as ambient background. For example, temperature gradients exist from the operation of a combustion engine and in urban areas, there is a large amount of electromagnetic energy in the environment because of radio and television broadcasting.
One of the earliest applications of ambient power collected from ambient electromagnetic radiation (EMR) is the crystal radio.
The principles of energy harvesting from ambient EMR can be demonstrated with basic components .
Operation
Energy harvesting devices converting ambient energy into electrical energy have attracted much interest in both the military and commercial sectors. Some systems convert motion, such as that of ocean waves, into electricity to be used by oceanographic monitoring sensors for autonomous operation. Future applications may include high power output devices (or arrays of such devices) deployed at remote locations to serve as reliable power stations for large systems. Another application is in wearable electronics, where energy harvesting devices can power or recharge cellphones, mobile computers, radio communication equipment, etc. All of these devices must be sufficiently robust to endure long-term exposure to hostile environments and have a broad range of dynamic sensitivity to exploit the entire spectrum of wave motions.
Accumulating energy
Energy can also be harvested to power small autonomous sensors such as those developed using MEMS technology. These systems are often very small and require little power, but their applications are limited by the reliance on battery power. Scavenging energy from ambient vibrations, wind, heat or light could enable smart sensors to be functional indefinitely. Several academic and commercial groups have been involved in the analysis and development of vibration-powered energy harvesting technology, including the Control and Power Group and Optical and Semiconductor Devices Group at Imperial College London, IMEC and the partnering Holst Centr, AdaptivEnergy, LLC, ARVENI, MIT Boston, Victoria University of Wellington, Georgia Tech, UC Berkeley, Southampton University, University of Bristol,[5] Micro Energy System Lab at The University of Tokyo, Nanyang Technological University,[6] PMG Perpetuum, ReVibe Energy, Vestfold University College, National University of Singapore,[7] NiPS Laboratory at the University of Perugia, Columbia University,[9] Universidad Autónoma de Barcelona and USN & Renewable Energy Lab at the University of Ulsan (Ulsan, South Korea). The National Science Foundation also supports an Industry/University Cooperative Research Center led by Virginia Tech and The University of Texas at Dallas called the Center for Energy Harvesting Materials and Systems.
Typical power densities available from energy harvesting devices are highly dependent upon the specific application (affecting the generator's size) and the design itself of the harvesting generator. In general, for motion powered devices, typical values are a few µW/cm³ for human body powered applications and hundreds of µW/cm³ for generators powered from machinery. Most energy scavenging devices for wearable electronics generate very little power.
Storage of power
In general, energy can be stored in a capacitor, super capacitor, or battery. Capacitors are used when the application needs to provide huge energy spikes. Batteries leak less energy and are therefore used when the device needs to provide a steady flow of energy.
Use of the power
Current interest in low power energy harvesting is for independent sensor networks. In these applications an energy harvesting scheme puts power stored into a capacitor then boosted/regulated to a second storage capacitor or battery for the use in the microprocessor [12] or in the data transmission. The power is usually used in a sensor application and the data stored or is transmitted possibly through a wireless method.
Motivation
The history of energy harvesting dates back to the windmill and the waterwheel. People have searched for ways to store the energy from heat and vibrations for many decades. One driving force behind the search for new energy harvesting devices is the desire to power sensor networks and mobile devices without batteries. Energy harvesting is also motivated by a desire to address the issue of climate change and global warming.
Devices
There are many small-scale energy sources that generally cannot be scaled up to industrial size:
- Some wristwatches are powered by kinetic energy (called automatic watches), in this case movement of the arm is used. The arm movement causes winding of its mainspring. A newer design introduced by Seiko ("Kinetic") uses movement of a magnet in the electromagnetic generator instead to power the quartz movement. The motion provides a rate of change of flux, which results in some induced emf on the coils. The concept is related to Faraday's Law.
- Photovoltaics is a method of generating electrical power by converting solar radiation (both indoors and outdoors) into direct current electricity using semiconductors that exhibit the photovoltaic effect. Photovoltaic power generation employs solar panels composed of a number of cells containing a photovoltaic material. Note that photovoltaics have been scaled up to industrial size and that large solar farms exist.
- Thermoelectric generators (TEGs) consist of the junction of two dissimilar materials and the presence of a thermal gradient. Large voltage outputs are possible by connecting many junctions electrically in series and thermally in parallel. Typical performance is 100-300 μV/K per junction. These can be utilized to capture mW.s of energy from industrial equipment, structures, and even the human body. They are typically coupled with heat sinks to improve temperature gradient.
- Micro wind turbine are used to harvest wind energy readily available in the environment in the form of kinetic energy to power the low power electronic devices such as wireless sensor nodes. When air flows across the blades of the turbine, a net pressure difference is developed between the wind speeds above and below the blades. This will result in a lift force generated which in turn rotate the blades. Similar to photovoltaics, wind farms have been constructed on an industrial scale and are being used to generate substantial amounts of electrical energy.
- Piezoelectric crystals or fibers generate a small voltage whenever they are mechanically deformed. Vibration from engines can stimulate piezoelectric materials, as can the heel of a shoe, or the pushing of a button.
- Special antennas can collect energy from stray radio waves, this can also be done with a Rectenna and theoretically at even higher frequency EM radiation with a Nantenna.
- Power from keys pressed during use of a portable electronic device or remote controller, using magnet and coil or piezoelectric energy converters, may be used to help power the device.
Ambient-radiation sources
A possible source of energy comes from ubiquitous radio transmitters. Historically, either a large collection area or close proximity to the radiating wireless energy source is needed to get useful power levels from this source. The nantenna is one proposed development which would overcome this limitation by making use of the abundant natural radiation (such as solar radiation).
One idea is to deliberately broadcast RF energy to power and collect information from remote devices:[13] This is now commonplace in passive radio-frequency identification (RFID) systems, but the Safety and US Federal Communications Commission (and equivalent bodies worldwide) limit the maximum power that can be transmitted this way to civilian use. This method has been used to power individual nodes in a wireless sensor network
Fluid flow
Airflow can be harvested by various turbine and non-turbine generator technologies. For example, Zephyr Energy Corporation’s patented Windbeam micro generator captures energy from airflow to recharge batteries and power electronic devices. The Windbeam’s novel design allows it to operate silently in wind speeds as low as 2 mph. The generator consists of a lightweight beam suspended by durable long-lasting springs within an outer frame. The beam oscillates rapidly when exposed to airflow due to the effects of multiple fluid flow phenomena. A linear alternator assembly converts the oscillating beam motion into usable electrical energy. A lack of bearings and gears eliminates frictional inefficiencies and noise. The generator can operate in low-light environments unsuitable for solar panels (e.g. HVAC ducts) and is inexpensive due to low cost components and simple construction. The scalable technology can be optimized to satisfy the energy requirements and design constraints of a given application.
The flow of blood can also be used to power devices. For instance, the pacemaker developed at the University of Bern, uses blood flow to wind up a spring which in turn drives an electrical micro-generator.
Photovoltaic
Photovoltaic (PV) energy harvesting wireless technology offers significant advantages over wired or solely battery-powered sensor solutions: virtually inexhaustible sources of power with little or no adverse environmental effects. Indoor PV harvesting solutions have to date been powered by specially tuned amorphous silicon (aSi)a technology most used in Solar Calculators. In recent years new PV technologies have come to the forefront in Energy Harvesting such as Dye Sensitized Solar Cells (DSSC). The dyes absorbs light much like chlorophyll does in plants. Electrons released on impact escape to the layer of TiO2 and from there diffuse, through the electrolyte, as the dye can be tuned to the visible spectrum much higher power can be produced. At 200 lux a DSSC can provide over 10 µW per cm².
Piezoelectric
The piezoelectric effect converts mechanical strain into electric current or voltage. This strain can come from many different sources. Human motion, low-frequency seismic vibrations, and acoustic noise are everyday examples. Except in rare instances the piezoelectric effect operates in AC requiring time-varying inputs at mechanical resonance to be efficient.
Most piezoelectric electricity sources produce power on the order of milliwatts, too small for system application, but enough for hand-held devices such as some commercially available self-winding wristwatches. One proposal is that they are used for micro-scale devices, such as in a device harvesting micro-hydraulic energy. In this device, the flow of pressurized hydraulic fluid drives a reciprocating piston supported by three piezoelectric elements which convert the pressure fluctuations into an alternating current.
As piezo energy harvesting has been investigated only since the late 1990s, it remains an emerging technology. Nevertheless, some interesting improvements were made with the self-powered electronic switch at INSA school of engineering, implemented by the spin-off Arveni. In 2006, the proof of concept of a battery-less wireless doorbell push button was created, and recently, a product showed that classical wireless wallswitch can be powered by a piezo harvester. Other industrial applications appeared between 2000 and 2005, to harvest energy from vibration and supply sensors for example, or to harvest energy from shock.
Piezoelectric systems can convert motion from the human body into electrical power. DARPA has funded efforts to harness energy from leg and arm motion, shoe impacts, and blood pressure for low level power to implantable or wearable sensors. The nanobrushes are another example of a piezoelectric energy harvester. They can be integrated into clothing. Multiple other nanostructures have been exploited to build an energy-harvesting device, for example, a single crystal PMN-PT nanobelt was fabricated and assembled into a piezoelectric energy harvester in 2016. Careful design is needed to minimise user discomfort. These energy harvesting sources by association affect the body. The Vibration Energy Scavenging Project is another project that is set up to try to scavenge electrical energy from environmental vibrations and movements. Microbelt can be used to gather electricity from respiration. Besides, as the vibration of motion from human comes in three directions, a single piezoelectric cantilever based omni-directional energy harvester is created by using 1:2 internal resonance. Finally, a millimeter-scale piezoelectric energy harvester has also already been created.
The use of piezoelectric materials to harvest power has already become popular. Piezoelectric materials have the ability to transform mechanical strain energy into electrical charge. Piezo elements are being embedded in walkways to recover the "people energy" of footsteps. They can also be embedded in shoes to recover "walking energy". Researchers at MIT developed the first micro-scale piezoelectric energy harvester using thin film PZT in 2005. Arman Hajati and Sang-Gook Kim invented the Ultra Wide-Bandwidth micro-scale piezoelectric energy harvesting device by exploiting the nonlinear stiffness of a doubly clamped microelectromechanical systems (MEMSs) resonator. The stretching strain in a doubly clamped beam shows a nonlinear stiffness, which provides a passive feedback and results in amplitude-stiffened Duffing mode resonance. Typically, piezoelectric cantilevers are adopted for the above-mentioned energy harvesting system. One drawback is that the piezoelectric cantilever has gradient strain distribution, i.e., the piezoelectric transducer is not fully utilized. To address this issue, triangle shaped and L-shaped cantilever are proposed for uniform strain distribution.
In 2018, Soochow University researchers reported hybridizing a triboelectric nanogenerator and a silicon solar cell by sharing a mutual electrode. This device can collect solar energy or convert the mechanical energy of falling raindrops into electricity.
Energy from smart roads and piezoelectricity
Brothers Pierre Curie and Jacques Curie gave the concept of piezoelectric effect in 1880. Piezoelectric effect converts mechanical strain into voltage or electric current and generates electric energy from motion, weight, vibration and temperature changes as shown in the figure.
Considering piezoelectric effect in thin film lead zirconate titanate PZT, microelectromechanical systems (MEMS) power generating device has been developed. During recent improvement in piezoelectric technology, Aqsa Abbasi (also known as Aqsa Aitbar, General secretory at IMS, IEEE MUET Chapter and Director Media at HYD MUN [40][41][42][43][44]) diffentiated two modes called and in vibration converters and re-designed to resonate at specific frequencies from an external vibration energy source, thereby creating electrical energy via the piezoelectric effect using electromechanical damped mass.[45][46] However, Aqsa further developed beam-structured electrostatic devices that are more difficult to fabricate than PZT MEMS devices versus a similar because general silicon processing involves many more mask steps that do not require PZT film. Piezoelectric type sensors and actuators have a cantilever beam structure that consists of a membrane bottom electrode, film, piezoelectric film, and top electrode. More than (3~5 masks) mask steps are required for patterning of each layer while have very low induced voltage. Pyroelectric crystals that have a unique polar axis and have spontaneous polarization, along which the spontaneous polarization exists. These are the crystals of classes 6mm, 4mm, mm2, 6, 4, 3m, 3,2, m. The special polar axis—crystallophysical axis X3 — coincides with the axes L6,L4, L3, and L2 of the crystals or lies in the unique straight plane P (class “m”). Consequently, the electric centers of positive and negative charges are displaced of an elementary cell from equilibrium positions, i.e., the spontaneous polarization of the crystal changes. Therefore, all considered crystals have spontaneous polarization . Since piezoelectric effect in pyroelectric crystals arises as a result of changes in their spontaneous polarization under external effects (electric fields, mechanical stresses). As a result of displacement, Aqsa Abbasi introduced change in the components along all three axes . Suppose that is proportional to the mechanical stresses causing in a first approximation, which results where Tklrepresents the mechanical stress and dikl represents the piezoelectric modules.
PZT thin films have attracted attention for applications such as force sensors, accelerometers, gyroscopes actuators, tunable optics, micro pumps, ferroelectric RAM, display systems and smart roads,[45] when energy sources are limited, energy harvesting plays an important role in the environment. Smart roads have the potential to play an important role in power generation. Embedding piezoelectric material in the road can convert pressure exerted by moving vehicles into voltage and current.
Smart transportation intelligent system
Piezoelectric sensors are most useful in Smart-road technologies that can be used to create systems that are intelligent and improve productivity in the long run. Imagine highways that alert motorists of a traffic jam before it forms. Or bridges that report when they are at risk of collapse, or an electric grid that fixes itself when blackouts hit. For many decades, scientists and experts have argued that the best way to fight congestion is intelligent transportation systems, such as roadside sensors to measure traffic and synchronized traffic lights to control the flow of vehicles. But the spread of these technologies has been limited by cost. There are also some other smart-technology shovel ready projects which could be deployed fairly quickly, but most of the technologies are still at the development stage and might not be practically available for five years or more.
Pyroelectric
The pyroelectric effect converts a temperature change into electric current or voltage. It is analogous to the piezoelectric effect, which is another type of ferroelectric behavior. Pyroelectricity requires time-varying inputs and suffers from small power outputs in energy harvesting applications due to its low operating frequencies. However, one key advantage of pyroelectrics over thermoelectrics is that many pyroelectric materials are stable up to 1200 ⁰C or higher, enabling energy harvesting from high temperature sources and thus increasing thermodynamic efficiency.
One way to directly convert waste heat into electricity is by executing the Olsen cycle on pyroelectric materials. The Olsen cycle consists of two isothermal and two isoelectric field processes in the electric displacement-electric field (D-E) diagram. The principle of the Olsen cycle is to charge a capacitor via cooling under low electric field and to discharge it under heating at higher electric field. Several pyroelectric converters have been developed to implement the Olsen cycle using conduction, convection, or radiation. It has also been established theoretically that pyroelectric conversion based on heat regeneration using an oscillating working fluid and the Olsen cycle can reach Carnot efficiency between a hot and a cold thermal reservoir.[54] Moreover, recent studies have established polyvinylidene fluoride trifluoroethylene [P(VDF-TrFE)] polymers and lead lanthanum zirconate titanate (PLZT) ceramics as promising pyroelectric materials to use in energy converters due to their large energy densities generated at low temperatures. Additionally, a pyroelectric scavenging device that does not require time-varying inputs was recently introduced. The energy-harvesting device uses the edge-depolarizing electric field of a heated pyroelectric to convert heat energy into mechanical energy instead of drawing electric current off two plates attached to the crystal-faces.
Thermoelectrics
Thermoelectric generator
In 1821, Thomas Johann Seebeck discovered that a thermal gradient formed between two dissimilar conductors produces a voltage. At the heart of the thermoelectric effect is the fact that a temperature gradient in a conducting material results in heat flow; this results in the diffusion of charge carriers. The flow of charge carriers between the hot and cold regions in turn creates a voltage difference. In 1834, Jean Charles Athanase Peltier discovered that running an electric current through the junction of two dissimilar conductors could, depending on the direction of the current, cause it to act as a heater or cooler. The heat absorbed or produced is proportional to the current, and the proportionality constant is known as the Peltier coefficient. Today, due to knowledge of the Seebeck and Peltier effects, thermoelectric materials can be used as heaters, coolers and generators (TEGs).
Ideal thermoelectric materials have a high Seebeck coefficient, high electrical conductivity, and low thermal conductivity. Low thermal conductivity is necessary to maintain a high thermal gradient at the junction. Standard thermoelectric modules manufactured today consist of P- and N-doped bismuth-telluride semiconductors sandwiched between two metallized ceramic plates. The ceramic plates add rigidity and electrical insulation to the system. The semiconductors are connected electrically in series and thermally in parallel.
Miniature thermocouples have been developed that convert body heat into electricity and generate 40μW at 3V with a 5 degree temperature gradient, while on the other end of the scale, large thermocouples are used in nuclear RTG batteries.
Practical examples are the finger-heartratemeter by the Holst Centre and the thermogenerators by the Fraunhofer Gesellschaft.
Advantages to thermoelectrics:
- No moving parts allow continuous operation for many years. Tellurex Corporation[60] (a thermoelectric production company) claims that thermoelectrics are capable of over 100,000 hours of steady state operation.
- Thermoelectrics contain no materials that must be replenished.
- Heating and cooling can be reversed.
One downside to thermoelectric energy conversion is low efficiency (currently less than 10%). The development of materials that are able to operate in higher temperature gradients, and that can conduct electricity well without also conducting heat (something that was until recently thought impossible ), will result in increased efficiency.
Future work in thermoelectrics could be to convert wasted heat, such as in automobile engine combustion, into electricity.
Electrostatic (capacitive)
This type of harvesting is based on the changing capacitance of vibration-dependent capacitors. Vibrations separate the plates of a charged variable capacitor, and mechanical energy is converted into electrical energy. Electrostatic energy harvesters need a polarization source to work and to convert mechanical energy from vibrations into electricity. The polarization source should be in the order of some hundreds of volts; this greatly complicates the power management circuit. Another solution consists in using electrets, that are electrically charged dielectrics able to keep the polarization on the capacitor for years. It's possible to adapt structures from classical electrostatic induction generators, which also extract energy from variable capacitances, for this purpose. The resulting devices are self-biasing, and can directly charge batteries, or can produce exponentially growing voltages on storage capacitors, from which energy can be periodically extracted by DC/DC converters.
Magnetic induction
Magnets wobbling on a cantilever are sensitive to even small vibrations and generate micro currents by moving relative to conductors due to Faraday's law of induction. By developing a miniature device of this kind in 2007, a team from the University of Southampton made possible the planting of such a device in environments that preclude having any electrical connection to the outside world. Sensors in inaccessible places can now generate their own power and transmit data to outside receivers.
One of the major limitations of the magnetic vibration energy harvester developed at University of Southampton is the size of the generator, in this case approximately one cubic centimeter, which is much too large to integrate into today's mobile technologies. The complete generator including circuitry is a massive 4 cm by 4 cm by 1 cm nearly the same size as some mobile devices such as the iPod nano. Further reductions in the dimensions are possible through the integration of new and more flexible materials as the cantilever beam component. In 2012, a group at Northwestern University developed a vibration-powered generator out of polymer in the form of a spring. This device was able to target the same frequencies as the University of Southampton groups silicon based device but with one third the size of the beam component.
A new approach to magnetic induction based energy harvesting has also been proposed by using ferrofluids. The journal article, "Electromagnetic ferrofluid-based energy harvester", discusses the use of ferrofluids to harvest low frequency vibrational energy at 2.2 Hz with a power output of ~80 mW per g.
Commercially successful vibration energy harvesters based on magnetic induction are still relatively few in number. Examples include products developed by Swedish company ReVibe Energy, a technology spin-out from Saab Group. Another example is the products developed from the early University of Southampton prototypes by Perpetuum. These have to be sufficiently large to generate the power required by wireless sensor nodes (wsn)but in M2M applications this is not normally an issue. These harvesters are now being supplied in large volumes to power wsn's made by companies such as GE and Emerson and also for train bearing monitoring systems made by Perpetuum. Overhead powerline sensors can use magnetic induction to harvest energy directly from the conductor they are monitoring.
Blood sugar
Another way of energy harvesting is through the oxidation of blood sugars. These energy harvesters are called bio batteries. They could be used to power implanted electronic devices (e.g., pacemakers, implanted biosensors for diabetics, implanted active RFID devices, etc.). At present, the Minteer Group of Saint Louis University has created enzymes that could be used to generate power from blood sugars. However, the enzymes would still need to be replaced after a few years.[69] In 2012, a pacemaker was powered by implantable biofuel cells at Clarkson University under the leadership of Dr. Evgeny Katz.[70]
Tree-based
Tree metabolic energy harvesting is a type of bio-energy harvesting. Voltree has developed a method for harvesting energy from trees. These energy harvesters are being used to power remote sensors and mesh networks as the basis for a long term deployment system to monitor forest fires and weather in the forest. Their website says that the useful life of such a device should be limited only by the lifetime of the tree to which it is attached. They recently deployed a small test network in a US National Park forest.[71]
Other sources of energy from trees include capturing the physical movement of the tree in a generator. Theoretical analysis of this source of energy shows some promise in powering small electronic devices. A practical device based on this theory has been built and successfully powered a sensor node for a year.
Metamaterial
A metamaterial-based device wirelessly converts a 900 MHz microwave signal to 7.3 volts of direct current (greater than that of a USB device). The device can be tuned to harvest other signals including Wi-Fi signals, satellite signals, or even sound signals. The experimental device used a series of five fiberglass and copper conductors. Conversion efficiency reached 37 percent. When traditional antennas are close to each other in space they interfere with each other. But since RF power goes down by the cube of the distance, the amount of power is very very small. While the claim of 7.3 volts is grand, the measurement is for an open circuit. Since the power is so low, there can be almost no current when any load is attached.
Atmospheric pressure changes
The change in air pressure due to temperature changes or weather patterns vs. a sealed chamber has been used to provide power for mechanical clocks such as the Atmos clock.
What is an Atmospheric pressure change?
An Atmospheric change is a force exerted on a surface by air above as gravity pulls it to Earth. This is often measured by the barometer, a slight mercury in the glass tube rises and drops depending on changes in altitude and pressure. Usually, atmosphere pressure drops as altitude increases, also vice versa. Atmospheric pressure consists of molecules in constant motion, colliding with each other about 1010 collisions per molecule per second at 10 °C (50 °F), this is part of the kinetic energy of the atmosphere. With given devices/technology (Wind Turbine) in the contemporary era, industries/individuals can harvest or convert atmospheric energy into Electrical energy with low-cost efficient or even free! Overall an Atmospheric pressure is a great utility that can be devised into our economy for top-tier energy harvest and future solution for many other issues.
Ocean Energy
A relatively new concept of generating energy is to generate energy from oceans. Large masses of waters are present on the planet which carry with them great amounts of energy.The energy in this case can be generated by tidal streams, ocean waves, difference in salinity and also difference in temperature. Efforts are underway to harvest energy this way as it holds a great potential and would be a renewable form of energy. United States Navy recently was able to generate electricity using difference in temperatures present in the ocean. The project didn't yielded that much energy but sure there is a lot of potential.
Another idea is to generate an artificial flow in ocean to generate energy. If a flow of considerable magnitude could be generated it would produce large amounts of energy.
Future directions
Electroactive polymers (EAPs) have been proposed for harvesting energy. These polymers have a large strain, elastic energy density, and high energy conversion efficiency. The total weight of systems based on EAPs(electroactive polymers) is proposed to be significantly lower than those based on piezoelectric materials.
Nano generators, such as the one made by Georgia Tech, could provide a new way for powering devices without batteries. As of 2008, it only generates some dozen nano watts, which is too low for any practical application.
Noise has been the subject of a proposal by NiPS Laboratory in Italy to harvest wide spectrum low scale vibrations via a nonlinear dynamical mechanism that can improve harvester efficiency up to a factor 4 compared to traditional linear harvesters.
Combinations of different types of energy harvesters can further reduce dependence on batteries, particularly in environments where the available ambient energy types change periodically. This type of complementary balanced energy harvesting has the potential to increase reliability of wireless sensor systems for structural health monitoring.
XXX . ___ . 000 Vibrational analysis for the nuclear–electronic orbital method
The methodology for a vibrational analysis within the nuclear–electronic orbital (NEO) framework is presented. In the NEO approach, specified nuclei are treated quantum mechanically on the same level as the electrons, and mixed nuclear–electronic wave functions are calculated variationally with molecular orbital methods. Both electronic and nuclear molecular orbitals are expressed as linear combinations of Gaussian basis functions. The NEO potential energy surface depends on only the classical nuclei, and each point on this surface is optimized variationally with respect to all molecular orbitals as well as the centers of the nuclear basis functions. The NEO vibrational analysis involves the calculation, projection, and diagonalization of a numerical Hessian to obtain the harmonic vibrational frequencies corresponding to the classical nuclei. This analysis allows the characterization of stationary points on the NEO potential energy surface. It also enables the calculation of zero point energy corrections and thermodynamic properties such as enthalpy, entropy, and free energy for chemical reactions on the NEO potential energy surface. Illustrative applications of this vibrational analysis to a series of molecules and to a nucleophilic substitution reaction are presented.
We have developed the nuclear-electronic orbital (NEO) method for the incorporation of nuclear quantum effects into electronic structure calculations. In the NEO approach, specified nuclei are treated quantum mechanically on the same level as the electrons, and mixed nuclear-electronic wavefunctions are calculated with molecular orbital techniques. For hydrogen transfer and hydrogen bonding systems, typically the key hydrogen nuclei and all electrons are treated quantum mechanically. This approach naturally includes nuclear quantum effects such as proton delocalization and zero point energy as well as non-Born-Oppenheimer effects between the electrons and quantum protons.
Electron-proton dynamical correlation is highly significant and challenging to describe because of the attractive electrostatic interaction between the electron and the proton. We have developed the following wavefunction methods within the NEO framework: perturbation theory (MP2),70 multiconfigurational self-consistent-field (MCSCF) and configuration interaction (CI),54 nonorthogonal configuration interaction (NOCI),75 and explicitly correlated methods with Gaussian-type geminal functions (XCHF and RXCHF).86, 103, 108, 142, 167, 192, 193 The explicitly correlated methods have also been successful in describing positron systems.100, 112, 154, 168, 220
The NEO method has been extended to treat multiple protons quantum mechanically125 and has been combined with the fragment molecular orbital (FMO) method123 to treat larger systems as well as with vibronic coupling theory111 to incorporate the effects of other vibrational modes in tunneling splitting calculations.
The most promising approach within the NEO framework is multicomponent density functional theory (NEO-DFT).96, 105, 118 Electron-proton correlation functionals have been developed based on the explicitly correlated electron-proton pair density105, 145, 154 and, more recently, based on the Colle-Salvetti formulation (the epc17 functionals).231, 232 NEO-DFT/epc17 has been shown to produce accurate proton densities231 as well as energetic quantities such as proton affinities and optimized geometries.232
Current theory and method development projects are focusing on developing more accurate electron-proton correlation functionals and developing NEO-TDDFT (time-dependent DFT) for computing excited electron-proton vibronic states. Understanding the fundamental nature of the nuclear quantum effects, such as zero point energy and proton delocalization, and non-Born-Oppenheimer effects is also of great interest.
Applications of interest include calculating pKa’s for molecular electrocatalysts; generating reaction paths for proton, hydride, and proton-coupled electron transfer reactions; calculating hydrogen tunneling splittings and vibronic couplings; and characterizing photoinduced proton-coupled electron transfer systems.
Non-destructive imaging of buried electronic interfaces using a decelerated scanning electron beam
Recent progress in nanotechnology enables the production of atomically abrupt interfaces in multilayered junctions, allowing for an increase in the number of transistors in a processor. However, uniform electron transport has not yet been achieved across the entire interfacial area in junctions due to the existence of local defects, causing local heating and reduction in transport efficiency. To date, junction uniformity has been predominantly assessed by cross-sectional transmission electron microscopy, which requires slicing and milling processes that can potentially introduce additional damage and deformation. It is therefore essential to develop an alternative non-destructive method. Here we show a non-destructive technique using scanning electron microscopy to map buried junction properties. By controlling the electron-beam energy, we demonstrate the contrast imaging of local junction resistances at a controlled depth. This technique can be applied to any buried junctions, from conventional semiconductor and metal devices to organic devices.
Non-destructive imaging of buried electronic interfaces using a decelerated scanning electron beam
Recent progress in nanotechnology enables the production of atomically abrupt interfaces in multilayered junctions, allowing for an increase in the number of transistors in a processor. However, uniform electron transport has not yet been achieved across the entire interfacial area in junctions due to the existence of local defects, causing local heating and reduction in transport efficiency. To date, junction uniformity has been predominantly assessed by cross-sectional transmission electron microscopy, which requires slicing and milling processes that can potentially introduce additional damage and deformation. It is therefore essential to develop an alternative non-destructive method. Here we show a non-destructive technique using scanning electron microscopy to map buried junction properties. By controlling the electron-beam energy, we demonstrate the contrast imaging of local junction resistances at a controlled depth. This technique can be applied to any buried junctions, from conventional semiconductor and metal devices to organic devices.
Introduction
Almost all electronic and spintronic devices1have junctions between different types of semiconductors (for example, p–n junctions) and metals and non-metals (for example, metal/semiconductor and tunnel junctions) at the core of their functionality. The efficiency and even the actual functionality of such devices are determined by the quality of the interfaces between the constituent layers. By definition, these interfaces in junction structures are buried beneath the surface layer and hence any defects, either in the form of pinholes or impurities, are impossible to be imaged without using time-consuming and complex techniques as summarized in Fig. 1. These techniques are based on microscopy, spectroscopy, scattering and reflection, and electrical methods. Microscopic techniques are the most powerful ones among them, such as cross-sectional transmission electron microscopy (TEM)2, and can reveal detailed information about atomic structures at the junction interfaces. However, the additional preparation required for a cross-sectional sample involves erosion and strain-induced damage of the junction and hence features that subsequently appear in the imaging process may be due to the sample fabrication process rather than being inherent in the original device. Helium ion microscopy (HIM) has also been used to observe the topology, while the helium ion beam can mill the sample in situ3. HIM can therefore achieve subsurface imaging on a semiconductor structure with sub-nm resolution but it is destructive to image buried junctions. To avoid such preparation processes, electron beam-induced current has been developed to image the distributions of the conductive properties of the buried junctions4. However, this technique is limited to conductive samples with the resolution of sub-micron and is difficult to make quantitative analysis on the junction properties, such as their conductivities and the position of the defects. For a semiconductor integrated circuit, voltage alteration techniques in scanning electron microscopy (SEM) can also be used but their resolution is typically in a micron scale5.

Major techniques for the evaluation of buried junctions against destructiveness and resolution, including those based on microscopy (closed circles), spectroscopy (closed squares), scattering and reflection (closed triangles), and electrical methods (closed inverse triangles). For the microscopic methods, (S)TEM and HIM denote (scanning) transmission electron microscopy and Helium ion microscopy. For the spectroscopic methods, SIMS, AES, EDX, CL, ATR-IR and XPS denote secondary ion mass spectroscopy, Auger electron spectroscopy, energy dispersive X-ray spectroscopy, cathode luminescence, attenuated total reflection-infrared spectroscopy and X-ray photoelectron spectroscopy, respectively. For the scattering and reflection methods, XRT, GISAXS, RBS and XRR represent X-ray topography, grazing-incident small-angle X-ray scattering, Rutherford backscattering and X-ray reflectivity, respectively. For the electrical methods, I–V indicates current–voltage measurements.
On the other hand, spectroscopic techniques can disclose the chemical composition in the vicinity of the junction interfaces and can be used in combination with other techniques, such as microscopic imaging. Secondary ion mass spectroscopy6, Auger electron spectroscopy7, energy dispersive X-ray spectroscopy and cathode luminescence8 have nanometric resolution but they require destructive sample preparation, typically Ar-ion bombardment, to expose the desired interfaces for analysis. By extending the penetration depth by employing different probing light or X-ray, attenuated total reflection-infrared spectroscopy9 or X-ray photoelectron spectroscopy) (http://srdata.nist.gov/xps/DataDefinition.aspx) can be used in a non-destructive way. However, these techniques superimpose all the information from the layers the beam penetrates through. These techniques have their resolution to be limited by their wavelength, that is, almost a sub-micron scale.
For junction evaluation techniques based on reflection and scattering, elipsometry has both nanometric resolution and non-destructiveness10. However, it requires an analytical model for fitting and is difficult to be correlated to transport properties. Rutherford backscattering11 and X-ray reflectivity12 have also been commonly used to evaluate the interfacial roughness microscopically, which again requires an analytical model for data fitting. To avoid such models, X-ray topography13 and grazing-incident small-angle X-ray scattering14 have recently been developed. These techniques still need more improvement, particularly in their resolution. In addition, a conventional current–voltage (I–V) measurement has been utilized to assess a macroscopic junction potential. For example, for a tunnel junction, Simmons’ fitting15 is typically used to estimate the barrier height and thickness. Such electrical methods are important to determine the device performance but they cannot reveal microscopic transport properties.
In this study, a decelerated electron beam was used to control its penetration depth into a multilayered junction. The beam generated secondary and backscattered electrons after impacting on a junction layer under evaluation. By combining the control of the electron-beam voltage and energy filters, these electrons generated at a specified layer were collected to produce an image of conductance distributions across the buried junction. Our technique can be used as a quality assurance tool in nanoelectronic device fabrication.
Results
Electron-beam deceleration technique
A field-emission scanning electron microscope (JEOL, JSM-7800F Prime) was used to develop a technique for potential mapping on buried junctions spanning a wide range of resistance. Here to maintain nanometric resolution, the initial electron beam was accelerated at (Vspec+Vacc) keV, where the bias voltage for specimen was −Vspec and the effective acceleration voltage landed on the specimen was Vacc. This ensures high-imaging resolution, ∼0.7 nm resolution for Vspec=5 keV, Vacc=1 keV for example. In our observation, we managed to identify defects with their diameter of a few nanometres clearly (Fig. 2b,c). The beam was then decelerated in the vicinity of the specimen stage (the so-called gentle beam mode). This enabled us to control the beam penetration depth to match the vertical position of the buried junction interface under evaluation and the resulting secondary electrons (SE) and backscattered electrons (BSE) to be generated near the buried junction interfaces. In combination with an energy filter placed beneath the upper electron detector (UED) and the upper secondary electron detector (USD) (see Fig. 2a), more precise selection of the SE and BSE energies was achieved as detailed in Methods.

(a) Proposed technique in this study for the junction evaluation by decelerating electron beam to control its penetration depth to meet the vertical position of the junction. The alignment of the upper electron detector (UED), the upper secondary electron detector (USD) and the energy filter used in this study is also shown. (b) Scanning electron microscopy (SEM) image taken by UED with the secondary electron (SE) mode at Vacc=1 keV, which can penetrate into 10 nm below the surface. False blue and orange colour is provided for Ni0.8Fe0.2 and Cu wires. (c) Corresponding SEM image taken at Vacc=5 keV, which can penetrate into 100 nm below the surface. (d,e) Interaction volume simulations to estimate the penetration depth for b,c. (f) SEM image taken by USD with the SE mode at Vacc=5 keV using energy filter below −500 V as. (g) Corresponding SEM image taken by UED with the backscattered electron (BSE) mode at Vacc=5 keV using energy filter above −500 V.
For SEM imaging, either SE, BSE or both has been commonly used. In the specimen, incident electrons are scattered inelastically and generate SE according to the energy loss during scattering events. Typically SE can be generated in the vicinity of the surface (approximately three times of the mean free path of SE, that is, around 3–10 nm depending on the materials). Emitted SE to a vacuum can then be detected to create a SEM image. At a decelerated electron-beam energy below a few 100 eV, the incident beam is easily scattered by the materials in the specimen, allowing the interactions between the beam and materials to occur within a few nanometres of the surface. The deceleration voltage between 500 eV and 5 keV has therefore been used in this study to avoid such scattering and to ensure the detection of electrons from the buried junctions.
In a junction, sharp and clean interfaces produce reproducible interfacial resistances. Such a junction may offer interfacial resistances ranging from a few pΩ m2 to μΩ m2 depending on the combinations at the junction interfaces, such as residual resists and oxides. For example, a spin–valve interface consisting of a ferromagnetic metal/non-magnetic metal/ferromagnetic metal multilayer typically shows an interfacial resistivity of over a few nΩ m2. However, defects, such as pinholes and impurities, show much smaller or larger resistivities, which can become of the order of a few pΩ m2 or larger than a few μΩ m2, respectively. These differences in resistivities generate a potential difference of a few eV up to a few keV between the different interfacial conditions. To detect such a potential difference, the electron beam needs to be decelerated effectively to the keV order and below. This deceleration voltage depends on the energy levels of SE and BSE from the buried interfaces to be observed. These energy levels can be simulated by using a well-established model .
Fresh junction imaging
For a lateral spin–valve device consisting of two 200 × 200 nm Ni0.8Fe0.2/Cu junctions, SEM images were taken at two distinct effective accelerated voltages (Vacc) of 1 and 5 keV as shown in Fig. 2b,c, respectively. Here the bottom 30-nm-thick Ni0.8Fe0.2 nanowires were fabricated using a combination of standard electron-beam lithography and a lift-off process-first, followed by top 70-nm-thick Cu wires (see Methods for more details). The device was then characterized by the conventional four-probe method as explained in Methods, confirming the resistance to be ∼5 nΩm2. As simulated in Fig. 2d,e, the effective voltage controls the depth profiles of the electron beam for imaging. This means the effective voltage of 1 keV allows the electrons to only penetrate into the surface Cu layer above the interface as shown in Fig. 2d, while that of 5 keV allows the electrons to penetrate through the Ni0.8Fe0.2/Cu junction (Fig. 2e). This clearly indicates that the effective electron-beam acceleration voltage in the vicinity of the specimen surface is one of the key parameters to determine the depth of the measurement positions for the buried junction imaging proposed in this study. This technique is much simpler than electric-field measurements using cross-sectional TEM imaging16 for example.
By comparing the two images in Fig. 2b,c, one can identify defects in the Cu nanowires, which can be seen as grey regions and black dots. The brightness in a SEM image created by SE is proportional to the number of generated SE in the specimen. The SE generation depends on the effects of surface morphology, specimen edges, acceleration voltages, atomic number of the specimen materials and charging-up on the specimen surface. Here the Cu and Ni0.8Fe0.2 wires are proven to have smooth surface without showing clear contrast from Fig. 2b. As seen in Fig. 2b,c, the edges of the device show bright contrast as expected. The two SEM images obtained at two different acceleration voltages did not show a clear difference in their general contrast. The device we observed was conductive and therefore should not induce any charging-up effect. We can hence exclude these effects and can conclude that the difference in the contrast indicates variance in the conductance across the specimen. The regions with darker contrast may therefore represent defects in the device, which generate fewer SE than the other majority regions. In Fig. 2b, some minor grey regions are seen in the Ni0.8Fe0.2 wires, indicating that the Ni0.8Fe0.2 wires have some defects near the surface. Figure 2b also shows that the junction regions do not have any contrast, confirming that the Cu wire has no defects near the surface. On the other hand, Fig. 2c shows some grey regions in the Cu wire, indicating that either within the Cu wire or the bottom interface of the Cu wire, that is, Cu/Ni0.8Fe0.2 and Cu/Si, has some defects. Such defects can also be seen at the junction regions. The size of the defects is measured to be between 10 and 100 nm. It should be noted that no grey regions are observed in the Ni0.8Fe0.2 wires, confirming the Ni0.8Fe0.2 wires have no defects at the Ni0.8Fe0.2/Si interface.
Another key parameter in this study is the energy filtering of SE and BSE from the specimen. For lateral spin-valve junctions, the voltage is applied at 1 and 5 keV, which controls the depth profile of imaging as discussed above. The energies of the generated SE and BSE then need to be selected to represent only the conductance difference in the buried junction. A similar study has been carried out on semiconductor p–n junctions17, confirming that the conductance difference induced by chemical potentials can be detected as SEM image contrast. To characterize the buried interfacial defects, more precise control of the electron energies to be detected for imaging is required, which can be carried out by an additional energy filtering at the detector and an additional decelerator attachment to the specimen stage and the control of the total layer thickness above the junctions18.
Figure 2f,g shows SEM images taken at Vacc=5 keV using energy filters below −500 V with the SE mode and above −500 V with the BSE mode, respectively. Since SE can be emitted typically within 3 and 10 nm deep from the surface, the SE image shows almost identical contrast as that in Fig. 2c. In the BSE image in Fig. 2g, the defects in the Cu wire are unambiguously observed. In particular, almost a quarter of the junction is found to be covered by defects, such as at the top-right and bottom-left corners in the left hand side junction, and the left edge in the right hand side one. These defects suggest the presence of the contaminations at the junction interfaces, since they are typically non-conductive and do not produce SE and BSE. By comparing Fig. 2b,f,g, the interfacial defects are only found at the bottom Cu interface. These results prove that BSE have high spatial resolution and are highly sensitive to such defects in a buried junction.
The giant magnetoresistance (GMR) behaviour of the device is shown in Fig. 3d, which confirms that spin-polarized electrons are successfully injected into the Cu wire and are efficiently detected by the Ni0.8Fe0.2wire. This is measured under the non-local geometry at a current of 100 μA with showing the magnetoresistance ratio of 1.7% at room temperature as shown in Fig. 3d. This is similar to the values reported in similar devices19, assuring the quality of the lateral spin–valve junctions. The junction has then been damaged during the following measurements, showing the resistance to be increased up to a few μΩ m2.

(a) Scanning electron microscopy (SEM) image of broken Ni0.8Fe0.2/Cu junctions taken by upper electron detector (UED) with the BSE mode at Vacc=2.5 keV using energy filter above −500 V. (b) Interaction volume simulations to estimate the penetration depth for a. (c) Simulation of the generated backscattered electrons from the Cu layer. (d) Magnetoresistance result of the Ni0.8Fe0.2/Cu junction before the breakdown.
Broken junction imaging
After the junction breakdown, we observed the buried Ni0.8Fe0.2/Cu junctions as shown in Fig. 3a. Here Vacc of 2.5 keV is used, resulting the penetration of the electron beam to be down to ∼25 nm below the Cu surface (Fig. 3b). The penetrated electrons generate BSE in the Cu wire within ∼10 nm from the surface as simulated in Fig. 3c. Figure 3a reveals that many defects are formed in the broken Cu/Ni0.8Fe0.2 junctions and a part of the Cu wire is pealed off at the edges of the junctions. This suggests that the top Cu wire may be detached from the bottom Ni0.8Fe0.2 wire after junction breakdown, which is supported by the transport measurement with showing μΩ m2 resistivity. The black dots observed in the junctions are defects, which may be formed by detached interfaces. Such detached interfaces contain voids, which cannot generate BSE and can be shown as dark contrast in a SEM image. Similar defects are also seen in a part of Cu wire. These regions with many defects may be the area that can be detached by further current applications as seen in the right hand side junction in Fig. 3a. In summary, we can conclude that the deceleration technique has the capability to reveal defects and damage within the buried junctions in combination with standard simulations on electron scattering. Such a simple and non-destructive technique can be applied for other junctions by combining and controlling the deceleration voltage and energy filtering. This technique is expected to offer quality assurance for a wide range of electronic devices, consisting of nanoscale junctions (see GaAs/Fe and Fe/MgO/Fe junctions in Supplementary Figs 1 and 2, respectively). As discussed in the Supplementary Discussion, these junctions also show some contrast in their SEM images with a decelerated beam, proving the validity of the non-destructive method as shown above. This may allow further stacking and miniaturization of junctions to sustain the advancement in their density and functionality.
Methods
Device fabrication
The lateral spin–valve devices were fabricated by conventional electron-beam lithography and lift-off processes on a Si substrate with a thermally oxidised layer on the surface20. Two Ni0.8Fe0.2 nanowires were designed to be 30 nm thick and 200 nm wide with different shapes at their ends (square and sharp) to induce a difference in their magnetization-reversal fields. They were patterned using electron-beam lithography (JEOL, JBX-6300FS) and were deposited using high vacuum (HV) sputtering (SP) system (Leybold, UNIVEX 350). After their lift-off, these wires were bridged by a Cu nanowire (70 nm thick and 200 nm wide) made by the same manner. Before the Cu deposition, the surface of the Ni0.8Fe0.2 wires were cleaned by Ar-ion milling for 10 s at 50 W to remove oxides and contamination. Electrical contacts to these wires were finally made by photolithography (EGV, Mask Aligner) and lift-off process after the deposition of Cr (10 nm)/Au (150 nm) layers using an e-beam evaporator (Leybold, UNIVEX 350).
Magnetotransport measurement
The transport properties of the lateral spin–valves were assessed by non-local magnetoresistance measurements with a dc reversal method. Here an electrical current of 100 μA and an external magnetic field of up to ±1.2 kOe along the Ni0.8Fe0.2 nanowires were used for the measurements at room temperature.
SEM observation
In the JSM-7800F Prime SEM, two detectors, an UED and an USD, are located above the lens and two additional detectors, a retractable backscattered electron detector and a lower electron detector, are installed just above the sample space . The UED becomes sensitive to reflected electrons from the specimen with energies above 10 eV when a bias voltage (V) is applied to the energy filter beneath, while the USD senses those below 1 eV. These detection modes with energy deceleration in the range between +500 V and −2 keV can be achieved coincidently. In addition, the acceleration voltage for the electron beam can be controlled between 10 and 30 keV. Based on such controllability, 0.7-nm resolution is guaranteed at the effective electron-beam landing voltages of both 1 and 5 keV at the specimen by the manufacturer.
XXX . ____ . 000 000 Honey Bee Colonies Remote Monitoring System
Bees are very important for terrestrial ecosystems and, above all, for the subsistence of many crops, due to their ability to pollinate flowers. Currently, the honey bee populations are decreasing due to colony collapse disorder (CCD). The reasons for CCD are not fully known, and as a result, it is essential to obtain all possible information on the environmental conditions surrounding the beehives. On the other hand, it is important to carry out such information gathering as non-intrusively as possible to avoid modifying the bees’ work conditions and to obtain more reliable data. We designed a wireless-sensor networks meet these requirements. We designed a remote monitoring system (called WBee) based on a hierarchical three-level model formed by the wireless node, a local data server, and a cloud data server. WBee is a low-cost, fully scalable, easily deployable system with regard to the number and types of sensors and the number of hives and their geographical distribution. WBee saves the data in each of the levels if there are failures in communication. In addition, the nodes include a backup battery, which allows for further data acquisition and storage in the event of a power outage. Unlike other systems that monitor a single point of a hive, the system we present monitors and stores the temperature and relative humidity of the beehive in three different spots. Additionally, the hive is continuously weighed on a weighing scale. Real-time weight measurement is an innovation in wireless beehive—monitoring systems. We designed an adaptation board to facilitate the connection of the sensors to the node. Through the Internet, researchers and beekeepers can access the cloud data server to find out the condition of their hives in real time.
Introduction
Insect pollinators are essential for terrestrial ecosystems and for many agricultural and farming businesses. They ensure the maintenance of certain environmental processes, such as plant reproduction. Bees are the most specialized insect pollinators due to their ability to transport and store pollen efficiently [1]. Thus, honeybees are not only important for their honey production but also for environmental balance, because they are essential to the pollination of the flowers of many crops [2].
In recent years, honeybee populations have experienced significant losses due to colony collapse disorder (CCD). The reasons for the CCD are still being debated. As a result, it is essential to obtain information to look for solutions to this syndrome . Thus, bee colony monitoring—registering the largest possible amount of data but preventing the effects of handling on beehives—is important. In this way, it will be possible to obtain highly reliable information. Remote monitoring systems meet the necessary requirements to turn into a significant tool for the monitoring of bee colonies.
The use of precision apiculture allows us to monitor the beehives for many possible reasons, such as research, information about the daily management of bees by beekeepers, and learning how to reduce the resources and time assigned to tasks without reducing production.
Beehive monitoring allows us to monitor different parameters, such as the temperature and humidity levels inside the beehives, as well as the weight, sounds, and gases produced, which can generate important information. For example, these data can inform us on whether the beehives are swarming based on the temperature, whether any action is required from the beekeeper, whether the bees are affected by any disease, or even whether the hives are moving. This last application is very useful in areas where beehives can be stolen .
Different technologies can be applied to monitor the hives . Reducing the cost and size of the sensors allows the possibility of deploying them in the countryside to extract information and collect data more easily. Initially, remote electronic monitoring of beehives had a mainly scientific application, allowing the monitoring of factors inside the hives, such as the temperature and the humidity, with minimally invasive methods. Nowadays, these innovations have begun to be adapted by beekeepers through practical applications that can provide remote information for the decision-making processes without having to inspect the beehives.
However, the monitoring of biological processes is not a trivial task, due to the fact that the behavior of the biological system and its reaction to human interaction is not predictable. The acquisition of data in industrial processes is a very common issue, and there are many commercial systems for such tasks. However, in the scope of biological processes, data acquisition becomes a complex task, especially in the case of honeybee hives, where any foreign object can turn into a threat to be neutralized by the bees.
In recent years, monitoring systems have greatly progressed due to the use of wireless-sensor networks (WSNs). A WSN is made up of embedded devices that can acquire data from different sensors, process them, and communicate with a computer with a cloud database. These devices are known as nodes or motes and are the main core of the Internet of Things (IoT). Humans have used WSNs in many daily life activities, such as agriculture [10]. They have been also used in health care, intelligent home monitoring, archeological monitoring system, and military and industrial applications .
WSNs are also beginning to be used to monitor honeybee colonies. The nodes of a WSN can be used to obtain data from the sensors monitoring the environmental conditions of a beehive (temperature, humidity, CO2, etc.) and even its weight . The nodes connect and communicate through a gateway that can send the data from the motes to a base for storage and processing. This has increased the features of the monitoring systems, leading to what is currently known as precision apiculture. WSNs are a well-known alternative for distributed and remote sensing. These kinds of networks offer strong potential for beekeepers, due to the fact that they meet several significant requirements: (i) they are a minimally invasive method due to the small size of the nodes and sensors; (ii) they can be operational in remote areas for a long period of time due to their low energy consumption; and (iii) they allow real-time monitoring.
This work presents a wireless monitoring system for honeybee hives. The system architecture allows easy deployment in the field and ensures easy scalability. We used commercial nodes but adapted them to obtain measurements from the hives. We also developed an adaptor board.
Related Works
The interest regarding continuous honeybee colonies monitoring began during the first years of the XX-th century. In 1914, Gates published data on the temperature of a beehive manually collected every hour for several days in 1907 [15]. In 1926, Dunham measured the temperature inside a beehive by means of thermocouples [16]. The technological development of the sensors and electronic data-acquisition systems has allowed the improvement of measurement processes. In this way, different types of monitoring methods have been used, from simple observation of the data in the hive to systems that are able to analyze those data . Meikle and Holst summarized some of the continuous monitoring methods in , stating for each method the author, the measured parameters (temperature, O2, CO2, relative humidity, weight, vibration, etc.), and each method’s length.
One of the first electronic systems to monitor bees was Apidictor . This system consisted of a low-pass filter for frequencies to detect the changes of sound that took place inside the hive up to two to three weeks before swarming.
In , Ferrari et al. analyzed sound recorded with a sound card in a computer and monitored the temperature and relative humidity by means of a datalogger. The sound was recorded through three omnidirectional microphones. In , Chen et al. described an imaging system located at the entrance to the hive to find out the number of times a bee entered and exited the hive. They used a tag attached to the bee’s body to identify the bee under study. In , Zacepins and Stalidzans monitored the wintering process of bees, measuring the temperature of the beehive. The data were sent to a computer for processing and to determine the status of the hive by means of the application of an algorithm. In , Heidinger et al. used a radio-frequency identification (RFID) system to find out the frequency and length of the nuptial flights of honeybee queens.
Meikle et al. used electronics balances connected to a 12-bit resolution datalogger to assess the evolution of the weight of a beehive. The system was powered by a solar panel. They recorded the weight of two hives hourly .
The improvement of the performance and size of the microcontrollers has allowed the development of low-cost beehive-monitoring systems based on Arduino®, Make®, Sparkfun®, and Adafruit® . An example of an Arduino-based monitoring system is that proposed by Sánchez et al. . The system stored the temperature and relative humidity data in a microSD memory card by means of an Excel database. The beekeeper needed to go to the beehive colony and download the content of the SD memory card for each hive to his/her laptop to be able to process those data later on.
In recent years, honeybee hive monitoring systems based on WSNs have been used. These systems have the advantage of being able to carry out a non-intrusive remote monitoring of the hive’s environmental conditions. In [27], Kviesis et al. developed a system with a main unit that communicated wirelessly with each node located in the hive, concurrently acting as an Internet gateway. The system monitored the temperature and relative humidity using an integrated SHT15 sensor. The collected data were sent by the main unit to a remote database server. In , Zacepins et al. described a temperature-monitoring system based on Raspberry Pi acting as an Internet gateway. The temperature sensors located in the hives connected to the Raspberry Pi via a one-wire network. The authors highlighted the low cost of the solution if just one Raspberry Pi were used for all beehives.
In , Murphy et al. used a WSN to monitor a hive colony and collect the most important information on the activity/environment within a beehive, as well as its surrounding area. Each beehive was monitored by means of two nodes, and each of the nodes was implemented through a Waspmote® by Libelium (Zaragoza, Spain). To monitor the honeybees in the hive, several heterogeneous sensors were used (temperature, CO2, pollutants, NO2, etc.). Due to the high number of sensors, two commercial expansion boards were used, one for each Waspmote. Each sensor was read at a frequency of six samples/day. The collected samples were stored on an SD card for backup and were broadcasted over a Zigbee network once every 24 h to the base station. The base station acted as a bridge, sending the data to the remote server over a 3G/GSM connection. This monitoring system was used by Murphy et al. to propose several algorithms to automatically detect changes in the hive and warn the beekeeper of potential colony threats . Based on the CO2 levels of the hive, an algorithm was proposed to predict weather conditions.
Kridi et al. developed a system based on the Arduino platform to measure the temperature of the beehive by means of the LM35 sensor . The data were sent wirelessly through an XBee-Pro module to a base station desktop PC connected to the Internet. The collected data were processed to find patterns that determined the thermal stress in the hive to detect potential absconding conditions.
Joe-Air et al. developed a system to monitor the frequency of bee activity to relate the activity to environmental factors . It was a wireless system that monitored the frequency of bee entrances and exits from the hive together with the temperature and humidity inside and outside the hive. According to the authors, the accuracy obtained from the frequency depended on the activity of the hive, with an average error of 10% and a maximum value of 20%.
System Architecture
Beehive-monitoring systems must meet several significant requirements: (i) they must use a minimally invasive method; (ii) they must be operational in remote areas for long periods of time, and (iii) they must allow real-time monitoring. In [33], Kviesis and Zacepins described different automatic monitoring–system architectures for real-time beehive monitoring, distinguishing their advantages and disadvantages. In our case, we have chosen a three-level hierarchical model based on wireless communication. It is an easily adaptable model for any geographic distribution of hives, is scalable at any of the three levels, and meets the requirements indicated above.
Figure 1 shows the general structure of the beehive-monitoring system, WBee, described in this work. At the lowest level, we can find the wireless nodes that monitor the hives and send the data in wirelessly using IEEE 802.15.4. At the intermediate level, managing the wireless networks of a group of beehives, we can find an industrial computer with 802.15.4 communication that also acts as the network’s coordinator. This computer contains an application that collects the information sent by the nodes of each beehive and stores them in a local database. The communication with the global-server database is executed through another communication network that allows for greater distances, such as 3G/GPRS, WIFI, or WIMAX. Lastly, the upper level contains the cloud-server database, which groups several beehives and contains a copy of the local database of each group of hives.
The local computer for each apiary can work in isolation, since it can work as a database server. This characteristic ensures easy deployment and scalability of the system. Thus, new apiaries can be debugged before being integrated into the system. This local computer at the intermediate level is an important difference from other wireless monitoring systems in which a sink mote does not store information and is only used as a gateway between the WSN and global database server. Later, a more detailed description of each part will be provided.
The 802.15.4 standard is framed within the area of the wireless personal area networks (WPANs), with low consumption and cost. As a result, it has increasingly gained relevance in the industrial domain. Standard 802.15.4 only defines the physical and media-access control layers. Our applications directly communicate with the MAC layer, as shown in Figure 2.
Standard 802.15.4 defines two types of topologies: peer-to-peer and star networks. The peer-to-peer topology allows each node of the network to communicate with any other node, provided that the node is within its range. The star topology allows the establishment of communication among the devices and a sole central node that acts as the network coordinator. The network coordinator is responsible for starting and finishing the connections.
For this apiary monitoring system, we chose a star topology. Thus, the local computer acts as the coordinator. All nodes installed in the beehives must be accessible for the coordinator. This restriction does not imply a problem, since the beehives are on field and as a result, there are no significant obstacles usually. The use of this topology simplifies the communications, as we do not need nodes acting as routers or a network layer for frame routing.
Each node has two unique identifiers—the radio module’s MAC address and a node identifier (nodeID)—that allow it to be located by the application, similar to a hive address. In this way, when a radio module is replaced, only the same nodeID needs to be selected in the node. The nodeID has a length of 16 bits and can select up to 65,536 beehives. The application in the local computer collecting the data sent by each hive only needs to know the nodeID. This application maintains a table with the MAC addresses and the nodeID. These tables are dynamically created when the network is initialized, when a node is connected, and so on.
Wireless Node
The wireless node, which will be described in detail in Section 4, is based on the Waspmote by Libelium , but with an additional adapting board especially designed for the connection of three humidity and temperature sensors and the interconnection of a scale.
The system is designed so that each beehive has its own wireless node and scale. Figure 3 shows a photograph of the system attached to the beehive. The system is protected with an IP65 box. The weighing scale display has been kept so that the beekeeper can check the weight of the hive onsite.
3.2. Local Data Server, and Supervisory Control and Data Acquisition System
Two applications are executed on the local computer located in each apiary: a supervisory control and data acquisition system (SCADA), which synchronizes, requests, and processes the data of the node in each beehive, and MySQL, the world’s most popular open source database [35]. MySQL is also executed in the cloud data server, and a replica of the local database of each hive is carried out. This server guarantees an extra level of security in the event of a communications failure, since it stores and sends the data acquired in each beehive to the cloud. The local computer is an embedded industrial computer, which shows a higher protection against environmental conditions, as it must be placed beside the beehives. The computer communicates directly with the coordinator node of the wireless network using a USB interface, as shown in Figure 4. We used Libelium’s “XBee USB-Serial gateway” module as the coordinator of the network and gateway [36].
The software used for the execution of the SCADA was developed under Laboratory Virtual Instrumentation Engineering Workbench (LabVIEW), an environment developed by National Instruments (Austin, TX, USA) that uses a graphic programming language. The SCADA communicates with MySQL using the LabVIEW SQL/ODBC library to store the collected information . The application has one simple user interface in which it is possible to control the data acquisition interval for the data in the nodes (Figure 5), and a user-friendly part is responsible for the communication with each of the nodes, the data processing, and their storage.
The operation of the SCADA application is shown in Figure 6. Firstly, certain parameters are set in the application, such as the initialization of the coordinator node. Then, the application waits for the measurement interval programmed by the user. Once the wait is over, the broadcast for the beginning of the data acquisition is sent to the nodes so that all acquired samples are correlated, and afterward, the samples are requested from each node. If no data have been received from a specific node, they are requested again up to three times.
Cloud Database Server
A replica of the local database of each beehive is carried out in the cloud database server. This server ensures an extra level of security, providing both a backup and access the information of the beehives and sending the collected data to the cloud. As a result, through this server, the beekeeper can access all data about the beehives from anywhere through the Internet and can also receive alarms or other actions required by the beehives with an automated analysis of these data. Figure 7 shows the temperature and humidity data of a beehive stored in the cloud database server. The battery level and information regarding whether there are errors in the data acquisition from node are also stored in this database.
Implementation of the Wireless Node
The wireless node replaces the data acquisition and processing designed to monitor the thermoregulatory capacity of the honeybee colonies in hives with open-screened bottom boards . A new node and a different system architecture have been designed. In the previous system, three LM35 temperature sensors were installed in different areas of each beehive together with two SHT15 sensors, which can measure the hive’s temperature and humidity. An SHT15 was placed on the upper side of the beehive by the hole leading to the air chamber located under the lid. The other SHT15 sensor was placed under the hive to measure the outer temperature and humidity, allowing it to compare these data with the temperature and humidity inside the beehive. This system was installed in 10 hives. Five of the hives had conventional closed bottom boards and the other five had open-screened bottom boards. The electronic system was based on the Arduino platform and stored the data in a microSD memory. The beekeeper had to manually copy the content of the microSD card into his/her laptop for it to be processed later on. Thus, a new system based on a wireless sensor network was designed so that the measurement data obtained by each node were transmitted to a local server and from there, to the cloud database server. In this way, the beekeeper can see and download the measurement data for temperature, relative humidity, and weight from the Internet and more importantly, do so non-intrusively, not interfering with the work conditions of the beehive.
The wireless node is based on the Waspmote mote by Libelium . We selected this mote because it has the necessary characteristics to implement the most suitable wireless node to monitor a beehive. Among these characteristics, the following are worth mentioning: ultra-low power (7 µA hibernation mode), allowing the connection of any sensor using any wireless technology to any cloud platform; the ability to program it on the air (OTA); encryption libraries (AES, RSA); and several peripherals that will be mentioned below. Another advantage is that it allows the connection of several radio modules, depending on the transmission distance. In this way, by connecting the most appropriate radio module, it is possible to use long-range technologies (3G/GPRS/LoRaWAN/LoRa/Sigfox/868/900MHz), and in the case of isolated beehives, medium-range technologies (ZigBee/802.15.4/Wi-Fi) and short-range technologies (RFID/NFC/Bluetooth 4.0). On the other hand, the Waspmote platform includes the Waspmote-IDE (integrated development environment) used to program Waspmote [38]. This IDE offers a series of function libraries to easily control the different peripherals, such as the microSD card, RTC, UART, and the digital I/O.
Murphy et al. [29] also used the Waspmote for the implementation of a heterogeneous WSN, which monitors the internal conditions of a beehive colony though a diverse set of sensors. Murphy et al. used the Plug & Sense! version of Waspmote and the sensors offered by Libelium for that version with the appropriate connectors. This version of Waspmote has a drawback: If many sensors of the same type and a weighing scale need to be connected through a serial interface RS-232, as in our case, it is necessary to use two Waspmotes per node, as done by Murphy et al. in her implementation. Thus, we have decided to use the standard version of Waspmote and develop an adapter board in order to connect all sensors through the digital I/O ports. This board also includes the adaptation circuit for levels RS-232 to 3.3V CMOS to connect the weighing scale to the Waspmote UART. The resulting wireless node will be called UcoBee. We will describe both the hardware and the software of the UcoBee wireless node below.
Hardware Description Node
This section will include a description of the hardware of the UcoBee wireless node, Version 1, shown in Figure 8.
The block diagram of the wireless node is shown in Figure 9. The following blocks can be distinguished: Waspmote module, XBEE PRO module, adapter board, microSD memory card, battery, and external power supply.
Power Supply and Battery Block
Due to the closeness of the main 230 VAC power supply to the beehive apiary, two power supply units, which will be mentioned below, were used. If this were not available, the node could receive its power supply through a system based on a solar panel.
For the operation of the system installed in each beehive, two power voltages were needed: on the one hand, a power supply of 5 VDC/1 A with a miniUSB connector to supply the power to the Waspmote and another one of 12 VDC/0.5 A for the weighing scale with a female jack connector. The weighing scale includes a rechargeable lithium-ion 6 V and 2500 mAh battery, and a 3.7 V/2300 mAh rechargeable lithium-ion battery was connected to the Waspmote. Both the Waspmote and the weighing scale included a control circuit for loading the battery with the power supply. In this way, the wireless node could continue operating even if there were a power outage. If this happened, the measurement data would be stored on the microSD card and later on, when the power returned, they would be transferred to the local computer. The addition of the batteries, together with the power supply through a solar panel, allow the set of the wireless node and the weighing scale to be used in areas where there is no available electricity network near the apiary.
Humidity and Temperature Sensors
In order to measure the humidity inside the beehives, three SHT15 sensors by Sensirion were used . They were chosen due to their excellent reliability and stability and their low power consumption. These sensors allow the measurement of temperature by means of a band-gap sensor and of the relative humidity through a capacitive sensor. The SHT15 sensor includes a 14-bit analog/digital converter (ADC) and a serial interface circuit. The ADC converts the signal generated by both sensors into a digital signal and transfers the results of the conversion through the serial interface. In this way, a better-quality signal, quick response, and better immunity to external disturbances (EMC) are obtained. Within the SHT1x family, the SHT15 is the sensor with a higher accuracy, providing an error of ±0.3 °C for temperature and ±2% for relative humidity.
With the Plug & Sense! Version of Waspmote by Libelium, we would have needed to use the 808H5V5 humidity sensor and the MCP9700A temperature sensor. These sensors are analog, so they would have needed to be connected to the analog channels of the ATmega1281. In order to obtain the highest possible resolution in the ADC of the microcontroller, Waspmote Plug & Sense! must include a signal amplification board. Regardless, due to the fact that the ADC of the ATmega1281 is a 10-bit converter, a worse resolution would be obtained; since SHT15 sensors include an internal 14-bit ADC and because they are beside the measurement sensor, a better signal-noise ratio is obtained. On the other hand, the cost of the node is reduced, as Waspmote Plug & Sense! is more expensive.
The reading of and request for data is executed through a serial interface based on I²C known as Sensibus, as shown in Figure 10. This interface uses two signals: SCK and DATA. SCK is used to synchronize the communication between the microcontroller and the SHT15 sensor. The DATA pin is used to transfer data to or from the sensor. The SHT15 generates the measurement data with the falling edge of SCK. Thus, the microcontroller needs to read the data bits in the rising edge of SCK. The implementation of the communication protocol has been implemented through software using two I/O pins of the microcontroller.
The output digital signal is internally calibrated by means of calibration coefficients programmed in an internal OTP memory in the chip. This increases the stability of the signal to the internal variations due to the changes in temperature.
To reduce cost and obtain a reliable and easy connection for the sensor, we designed a PCB in which the SHT15 has been mounted and a male connector with a block device to connect it to the expansion board has been included, as can be seen in Figure 11a. Due to the tendency of bees to cover any foreign object inside their hive with propolis (resinous material collected by bees from bugs from trees and used as a cement to repair and maintain the hive), the sensors have been protected by enclosing them in perforated queen expedition cages Nicot®, as shown in Figure 11b.
Weighing Scale
The weighing scale consists of a metallic 50 cm × 40 cm frame with a 150 kg load cell associated. This weight is more than enough, as the weight of a beehive can be up to 80 kg. The load cell is connected to a BR80 display by Baxtran . The display has a six-digit screen, so it can display up to 100,000 different values. The resolution can be set up from 1 kg to 5 g. In our case, we have selected a resolution of 100 g, as we considered it to be sufficient for later analysis of the measurements, so that it is possible to determine, for example, whether the blooming period (and as a result, the bees’ honey production) is over.
The BR80 display has a DB9 connector that periodically sends the weight values through a series RS-232 interface, according to Figure 12. The frame consists of seven bytes. The first character sent is “=”, which is used to synchronize with the receiver (Waspmote) and to note that the six digits regarding the value of the weight will be then sent. The first digit is most significant, and as specified by standard RS-232, all of the characters are codified in ASCII code. The transmission speed can be configured from 1200 to 9600 bauds. The lowest speed has been selected in our case to allow the Waspmote to receive the measurements from the three SHT15 sensors without overflow occurring in the reception buffer of its UART.
Waspmote Module
The most important component of the wireless node is the Waspmote mote by Libelium. This mote is based on the ATmega1281 microcontroller by Atmel (San Jose, CA, USA). The ATmega1281 includes a 128 kB FLASH EPROM for the memory of the program, 8 kB SRAM to store data, a module to mount a microSD card, and a Real Time Clock (RTC). The size of the Flash memory is more than enough to store the application that controls the node, just as happens with the SRAM to temporarily store the data obtained by the sensors. In case more storage capacity is necessary, the microSD card can be used.
The Waspmote has a built-in RTC. The RTC we chose was the DS3231SN by Maxim (San Jose, CA, USA) which operates at a frequency of 32.768 Hz. This RTC can be programmed to generate an alarm to collect the values of the sensors and execute other actions, such as sending the values of the measurement data to the local server. Also, it allows Waspmote to use energy-saving modes and allows it to wake up at the required moment.
Another advantage of the Waspmote is that it has several expansion connectors. The adapter board, which will be described in a later section, will be connected to these expansion connectors. The Waspmote allows three power supply options: battery (3.3–4.2 V), USB connector (5 V), and solar panel (6–12 V @ 280 mA). When in operation, its consumption is 17 mA, whereas in the sleep mode, it is 33 µA, although due to the closeness of the mains to the colony, the power consumption does not represent a problem. Otherwise, the battery and the solar panel to charge the battery could be used, as was mentioned above.
An XBee-802.15.4-Pro module [41] was installed on the radio socket, with a maximum power consumption during transmission of 100 mW and a transmission frequency of 2.4 GHz. If a 5 dBi antenna is connected, its range reaches 7 km, allowing for perfect communication with the local server, as this is usually at a distance of no more than 20 m. If the bee colony had to be placed in a rural area far from the local server, a long-range radio module, such as GSM/GPRS, LoRaWAN, or LoRa, could be used.
Waspmote has a built-in LIS3331LDHS acceleration sensor by STMicroelectronics (Geneva, Switzerland), which informs the mote of acceleration variations experienced on each one of the three axes (X, Y, Z). As a result, it is possible to know whether anyone has moved the beehive or even worse, if anyone has tried to steal it. On the other hand, it is possible to connect an optional module with a GPS receiver to a Waspmote, which allows the beekeeper to know the exact outer location of the mote at any time. These two options were not used in the current application, but we are planning to include them in a later version to find out whether the beehive has been stolen and to know its exact location when searching for it. The accelerometer and the GPS module also affected the final choice of the Waspmote for the implementation of the wireless node.
Adapter Board
This board has been designed to be able to connect the three SHT15 temperature and humidity sensors and the RS-232 transmission serial signal generated by the weighing scale. Four connectors 4-way of 2 mm pitch for PCB assembly in right angle are used. These ensure a safe connection of the cable to the board, as they have a locking mechanism. The cable used for the connection is a twisted pair cable with sheet jacket by Alpha Wires (Elizabeth, NJ, USA), and the conductors are stranded wire tinned copper 24 AWG cables.
As mentioned, another function carried out by the adapter board is to adapt the RS-232 levels of the weighing scale’s transmission signal to the UART of the Waspmote, which is 3.3 V CMOS. The weighing scale does not include a RS-232 compatible driver, but it generates the signal with an operational amplifier supplied with a unipolar supply voltage of 10 V. Thus, if the transmission signal is idle or represents a data bit with value 1, it has a value of 0 V, but if it begins to transmit the start bit, or if it represents a data bit with value 0, it has a value of 8 V. As a result, the transmission signal meets the RS-232 standard for value 0, which corresponds to a voltage higher than +3 V. The fact that value 1 is represented by means of a voltage of 0 V does not imply any problem in our design, even though it does not meet standard RS-232, which states that the voltage must be lower than −3 V. On the contrary, as will be explained below, it will allow us to simplify the adapter circuit.
As Waspmote uses a supply voltage of 3.3 V, the reception input voltage range of the UART varies from 0 V to 3.3V. Therefore, the transmission signal of the weighing scale (8 V) cannot be directly connected to the UART (3.3 V max.). To adapt the voltage levels, the circuit shown in Figure 13 was designed. It has two bipolar transistors which, besides reducing the voltage from 8 V to 3.3 V approximately, inverts the logic value to meet the RS-232 standard.
To simplify the analysis, let us suppose that the Q2 transistor is saturated. Then, its function will be exposed and its operation will be analyzed. If the TXD_SCALE signal is at 0 V (idle or data bit with value 0), it does not drive transistor Q1. Thus, RXD_UART will be at a high logic level (3.3 V) through resistance R1. If, to the contrary, TXD_SCALE is at 8 V (start bit or data bit with value 1), the transistor gets saturated, and RXD_UART will be at a low logic level.
As the weighing scale periodically transmits the value of the weight every second and our application takes weight into consideration every 5 min, another transistor in a totem-pole mode was connected with the previous one. The base is controlled through a digital output of the Waspmote, which acts as reception enable. If ENA_RXD is at a low logic level, the Q2 transistor will be cut off. Then, independently from the state of the Q1 transistor, RXD_UART will be at a high logic level through R1 resistance. If on the other hand, ENA_RXD is active at a high logic level, the Q2 transistor gets saturated and the value of RXD_UART will depend on TXD_SCALE, as previously mentioned. In this way, the power consumption is reduced, and since Q2 is cut off when the Waspmote is not reading the weight data, there will be a high resistance between GND and the positive pole of the supply voltage, despite the saturation of Q1.
As previously mentioned, the Waspmote includes a microSD card. This is used to store the data regarding the measurements of the sensors and the weighing scale if communication with the local server is lost. In the expansion board, a switch, connected to a digital input of the Waspmote, has been included to enable the writing operation and to inhibit it, in order to remove it safely.
Additionally, a connector of the same type as those used to connect the sensors and the weighing scale has been added to the adaptor board. This connector is connected to the Waspmote microcontroller’s two analog channels. In this way, it will be possible to connect two analog output sensors (e.g., the CO2 sensors or a microphone to record sound). This connector can be power supplied if a signal amplification of the sensors should be necessary.
Software Node
The application executed in the wireless node was developed using the IDE-Waspmote open source-based environment. C language is used and several libraries are provided by the manufacturer. As in all Arduino environments, the application has a SETUP and a loop, which is indefinitely executed.
Once the node has been initialized and associated with the wireless network, the application is in standby, waiting for the reception of wireless orders. If no communication is received, the node collects samples using either the last programmed period or a default one and stores the samples on the microSD card. If there are no communication problems, the node will wait for the order to collect samples. In this way, we managed to get all network nodes to collect the samples at the same time. Then, the node waited for its samples to be requested. The node always works in this way as a slave of the computer in a master/slave model. The node has an RTC, which is synchronized with each reception-request packet. In each packet sent by the node with the collected data, the timestamp of its acquisition is included.
The RTC is necessary for the node to continue the data acquisition—even though there is no communication, as mentioned above—as together with the data, the time when the samples are acquired is also saved in the microSD card.
Figure 14 shows the flowchart of the application executed in the node. In normal operation mode, the node waits until it receives a broadcast sent by the local computer to collect the samples. Then, the node remains in standby, waiting to receive a new packet requesting the collected samples and responds with the samples and the timestamp.
Power Consumption and Autonomy of the Node
Each wireless node has its own backup battery. In normal conditions, the node is powered from another source of energy (solar panel or power grip). The Waspmote includes a control circuit for loading the battery with the power supply. This section describes the autonomy of the UcoBee node when the main power fails. A study of power consumption of the node will be carried out.
Table 1 shows the consumption indicated by the manufacturers. Since the consumption of SHT15 sensors is indicated for a 5 VDC supply, the consumption for 3.3 VDC is expected to be lower. The average consumption of the SHT15 indicated in the table is obtained by taking a measure per second.
Table 1
Power consumption according to manufacturer’s data.
Component | Operation Mode | Supply Current (Max) | Voltage |
---|---|---|---|
SHT15 | Sleep | 1.5 μA | 5 VDC |
Measuring | 1 mA | ||
Average | 28 μA | ||
Xbee Pro | idle/receive | 55 mA | 3.3 VDC |
Power down | <10 μA | ||
Transmission | 250 mA | ||
Waspmote | On | 17 mA | 3.3 VDC |
Deep sleep | 33 μA | ||
Hibernation | 7 μA |
The moment of acquisition and RTC of the nodes are synchronized with the local server, so the node can be kept in sleep mode and awakened before the acquisition request. This is possible using the RTC included in the Waspmote. Because physical magnitudes vary slowly over time, a 5-min minimum period of sampling has been considered, although the SCADA program that runs on the local server can be configured between 5 and 15 min. In order to reduce the power consumption of the node, once the measures have been acquired and sent to the local server, it goes into sleep mode for 4 min, so the node is in active mode for about a minute. Once the node is awakened, it performs the following operations: (1) Wait for the acquisition request, so the Xbee module is in reception mode; (2) Acquire the data from the SHT15 sensors; (3) Read the weight of the scale; and (4) Transmit the data to the local server, so the Xbee module is in transmission mode, and finally, the node goes into sleep mode.
It is necessary to distinguish two cases for the power consumption in active mode since there is a small difference in power consumption depending on whether or not the node has lost communication with the local server. The power consumption is higher when the Xbee Pro module is in transmission mode. If the communication is lost, the data is stored on the microSD card and the Xbee module does not transmit. In this situation, consumption is slightly lower.
Table 2 shows the experimental power consumption of the node. The measurements were performed on the network installed in the laboratory that was used to test the system. The network consisted of three nodes and a local database server. The measurements were performed with the active node acquiring data continuously and sending them to the local server. In the first two cases, the node did not transmit the acquired data, with the Xbee module remaining in receive mode. For the latter case, the nodes transmitted the data acquired continuously. The measurements were made observing the current consumption of the three nodes and calculating the average value. As we discussed in this paper this is not the average consumption in our application because the node is in sleep mode at least 80% of the time.
Table 2
Measured power consumption of the node.
Mode | Operation | Supply Current |
---|---|---|
Sleep | Sleep | 45 µA |
Active with communication | Measuring SHT15 | 70.5 mA |
Reading scale | 70.2 mA | |
Transmitting | 271.1 mA |
One of the most important aspects of the installation of the sensors network deployed in beehives is to know the battery autonomy when there is an outage in the power supply. To measure the autonomy of the nodes the main power to ten nodes installed in the apiary was removed for 75 h and the local database server was shut down. During that time, the sequence of operations is similar to the one described above, except that the Xbee module does not transmit data and the samples are stored on the microSD card. With a rechargeable lithium-ion battery of 3.7 V/2300 mAh, the battery level of the ten nodes that were analyzed fell an average of 70%, as shown in Figure 15. This time of autonomy is enough to solve the problem since the global server of the Wbee system generates an alarm when losing communication. Therefore it was not necessary to install a battery with higher capacity.
6. System Installation and Results
This system is being used in 20 Apis mellifera iberiensis bee colonies located in an experimental apiary in the University of Córdoba (Córdoba. Spain; 37°55′33.5′′ N, 4°43′26.1′′ W). A node has been installed on each beehive. The colonies were formed during spring 2016. The bees were housed in Langstroth beehives placed on supports 50 cm over the floor, as shown in Figure 16.
Three SHT15 temperature and relative-humidity sensors were installed in each beehive in different locations: (i) middle of the brood area; (ii) area with honey/pollen reserves in the periphery of the same brood comb; and (iii) honeycombs separated from the brood combs. All of the sensors were located 12 cm below the top of the comb, as shown in Figure 17a,b. A node with just one SHT15, protected from environmental conditions, was installed to measure the temperature and humidity outside the hives. Each beehive was installed on a scale connected to the wireless node to weigh it in real time. The wireless node and the display of the weighing device were protected inside an IP65 box by each beehive. Three sensors were installed because according to studies carried out they show significant variations in relation to the thermal regulation that the bees carry out in different zones of the hive . Thus, in the central brood area of the colony the temperatures are stable between 34–35 °C, which is the ideal temperature for the development of the bee larvae. However, as we move away from the brood area, temperatures are becoming less stable and therefore it is more similar to those outside the hive.
The local data server is correctly protected in a portable hut with an electric power supply. The cloud data server was installed in a building of the University Campus of Rabanales at the University of Cordoba.
Although the system began testing in September 2015, we chose to monitor the response of the bees to a sunflower bloom at the beginning of summer 2016, between 1 June and 2 July. The temperature, humidity, and weight in the hives were registered every 5 min.
WBee provided a large amount of very useful experimental data for researchers and beekeepers. As an example, three graphs of the average evolution of four beehives during the evaluation of the hives during the sunflower bloom are shown below.
Figure 18 shows the average evolution of the weight of four hives over 32 days. From Day 26 onward, it is possible to see that the weight of the beehives stabilizes, and this is interpreted as the end of the blooming period, implying that the beekeeper can now remove the honey harvest from the hives.
Figure 19 shows the temperature data registered during the experiment (32 days). The data show the ambient temperature outside the beehives (AT) and temperatures recorded in different areas of the beehives: (T1) middle of brood area; (T2) area with honey/pollen reserves in the periphery of the same brood comb; and (T3) honeycombs separated from the brood combs. The data correspond to the daily average temperature of four hives. Environmental temperature affected the temperatures inside the beehive, although it has been noted that the sensors placed in the middle of the brood area recorded higher and more stable mean temperatures within the hives.
Average temperature per day of four hives over 32 days. Temperatures were registered at ambient (AT) and three areas inside the beehives: brood area (T1), area with honey/pollen reserves of in the periphery of the brood comb (T2), and in honeycombs (T3).
Figure 20 shows the ambient humidity outside the beehives (AH) and humidity recorded in different areas of the beehives: (H1) middle of brood area; (H2) area with honey/pollen reserves in the periphery of the same brood comb; and (H3) honeycombs separated from the brood combs. The data correspond to the daily average humidity of four hives. The humidity inside of the beehive stays more stable than the outer humidity.
Conclusions
o:We have designed a low-cost, reliable beehive-monitoring system based on a WSN to measure the temperature, relative humidity, and weight of beehives in real time and non-intrusively. Unlike other beehive-monitoring systems, WBee performs the synchronized acquisition of samples from all hives of an apiary. This aspect is fundamental for future analysis of the data and their comparison between different hives. It will also allow researchers to compare physical magnitudes that change value quickly. Real-time weight measurement of the hive is an innovation in wireless beehive-monitoring systems.
WBee saves the data in each part of the network if there are failures in communication. In addition, the nodes include a backup battery for further acquisition and storage of data in the event of a power outage that could be sent once communication is reestablished. The use of a local database server is a novelty respect to other honeybee wireless monitoring systems. Other systems use a sink mote from the node manufacturer, which only works as gateway, and thus it does not maintain the database synchronized with the cloud database server. WBee obtains more information on the conditions of the hives than other systems because it monitors the temperature and relative humidity of the beehive in three different spots.
We managed to use just one Waspmote node per hive, as we designed an adapter board for the connection of the SHT15 sensors and RS232 interface of the weighing scale. The adapter board includes a simple adaptation circuit of RS232 levels, which reduces the power consumption of the node when the weight data is not desired.
Using a rechargeable lithium-ion battery of 3.7 V/2300 mAh, a minimum autonomy of 75 h is achieved. This is enough time to solve the problem since the global server of the Wbee system generates an alarm when losing communication. Greater autonomy can be achieved by increasing the acquisition period.
The system is scalable regarding the number of nodes and physical-measurement parameters. The architecture used to implement the system allows the data of all colonies to be accessible on the Internet through one unique cloud database server. It is also possible to access the data of a beehive on the Internet by connecting to the local database server.
We have been able to prove the reliability of the WBee system without affecting the precision of the measurements, against the propolis-covering action of the bees and the environmental conditions of the area where the experiment was developed, in low temperatures in winter (especially during the night), and in high temperatures during the rest of the year, above all, in summer.
The system was installed beginning in September 2015, providing data to a vet team from the University of Córdoba of the AGR-218 Research Group (Improvement and Maintenance of Genetic Resources of Domestic Animals, Apiculture Unit) for the study of the relationships between the bee colonies and the environment and of their management by beekeepers. The analysis of the data has allowed us to check the ability of honeybees to regulate temperature and humidity under tested conditions, as well as how beekeepers handle the beehives affects those conditions and their relationship with the health of the hives and their production.
The measurement of the weight of the hives has allowed us to understand the evolution of the bee colonies during blooms, in particular, during a commercial sunflower bloom, as well as its practical application in usual management by beekeepers. As an example, it allows us to register the evolution of the production of honey in the hives or the end of the bloom and estimate the production of honey or indicate the most appropriate moment for the collection of the honey in advance, preventing unnecessary trips to the apiary by the beekeeper.
XXX . ____ . 000 000 101010 ELECTRON ENERGY TRANSPORT IN BIO PLANTS
In with One Energy and out with Another
The light-dependent reactions take place in the thylakoid membrane, inside chloroplasts. Since they are light 'dependent' reactions, you can guess that these reactions need light to work. Remember that the purpose of this first part of photosynthesis is to convert sunlight energy into other forms of energy?
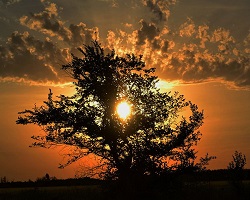
The light-dependent reactions of photosynthesis require sunlight. Image by Mell27.
Plants cannot use light energy directly to make sugars. Instead, the plant changes the light energy into a form it can use: chemical energy. Chemical energy is all around us. For example, cars need the chemical energy from gasoline to run. The chemical energy that plants use are stored in ATP and NADPH. ATP and NADPH are two kinds of energy-carrying molecules. These two molecules are not only in plants, as animals use them as well.
A Recipe for Energy
Plants need water to make NADPH. This water is broken apart to release electrons (negatively charged subatomic particles). When water is broken it also creates oxygen, a gas that we all breathe.
The electrons must travel through special proteins stuck in the thylakoid membrane. They go through the first special protein (the photosystem II protein) and down the electron transport chain. Then they pass through a second special protein (photosystem I protein).
Photosystem I and Photosystem II
Wait a second... first electrons go through the second photosystem and second they go through the first? That seems really confusing. Why would they name the photosystems that way?
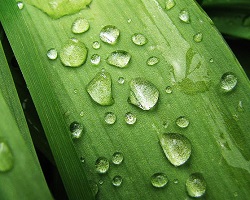
Water molecules are broken down to release electrons. These electrons then move down a gradient, storing energy in ATP in the process. Image by Jina Lee.
Photosystem I and II don't align with the route electrons take through the transport chain because they weren't discovered in that order.
Photosystem I was discovered first. Later, photosystem II was discovered and found to be earlier in the electron transport chain. But it was too late, the name stuck. Electrons first travel through photosystem II and then photosystem I.
Photosystem I was discovered first. Later, photosystem II was discovered and found to be earlier in the electron transport chain. But it was too late, the name stuck. Electrons first travel through photosystem II and then photosystem I.
The Electron Transport Chain
While at photosystem II and I, the electrons gather energy from sunlight. How do they do that? Chlorophyll, which is present in the photosystems, soaks up light energy. The energized electrons are then used to make NADPH.
The electron transport chain is a series of molecules that accept or donate electrons easily. By moving step-by-step through these, electrons are moved in a specific direction across a membrane. The movement of hydrogen ions are coupled with this. This means that when electrons are moved, hydrogen ions move too.
ATP is created when hydrogen ions are pumped into the inner space (lumen) of the thylakoid. Hydrogen ions have a positive charge. Like in magnets, the same charges repel, so the hydrogen ions want to get away from each other. They escape the thylakoid through a membrane protein called ATP synthase. By moving through the protein they give it power, like water moving through a dam. When hydrogen ions move through the protein and down the electron transport chain, ATP is created. This is how plants turn to sunlight into chemical energy that they can use.
The electron transport chain is a series of molecules that accept or donate electrons easily. By moving step-by-step through these, electrons are moved in a specific direction across a membrane. The movement of hydrogen ions are coupled with this. This means that when electrons are moved, hydrogen ions move too.
ATP is created when hydrogen ions are pumped into the inner space (lumen) of the thylakoid. Hydrogen ions have a positive charge. Like in magnets, the same charges repel, so the hydrogen ions want to get away from each other. They escape the thylakoid through a membrane protein called ATP synthase. By moving through the protein they give it power, like water moving through a dam. When hydrogen ions move through the protein and down the electron transport chain, ATP is created. This is how plants turn to sunlight into chemical energy that they can use.
The Calvin Cycle: Building Life from Thin Air
How does something like air become the wood of a tree? The answer lies in what makes up the air.
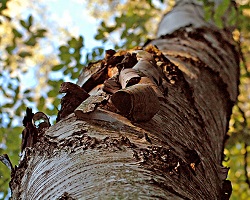
How can the air surrounding a tree be turned into tree material? Through a complex set of reactions that use the carbon from the air to make other materials. Image by André Karwath.
The air holds different elements like oxygen, carbon, and nitrogen. These elements make up molecules like carbon dioxide (CO2). Carbon dioxide is made out of one carbon atom and two oxygen atoms. Plants take the carbon atom from carbon dioxide and use it to build sugars.
This is done using the Calvin cycle. The Calvin cycle occurs inside chloroplasts, but outside the thylakoids (where ATP was created). The ATP and NADPH from the light-dependent reactions are used in the Calvin cycle.
Parts of the Calvin cycle are sometimes called light-independent reactions. But don't let the name fool you... those reactions do require sunlight to work.
The protein RuBisCO also helps in the process to change carbon from the air into sugars. RuBisCO works slowly, so plants need a lot of it. In fact, RuBisCO is the most abundant protein in the world!
The products of the Calvin cycle are used to make the simple sugar glucose. Glucose is used to build more complex sugars like starch and cellulose. Starch stores energy for the plant and cellulose is the stuff of which plants are made.
This is done using the Calvin cycle. The Calvin cycle occurs inside chloroplasts, but outside the thylakoids (where ATP was created). The ATP and NADPH from the light-dependent reactions are used in the Calvin cycle.
Parts of the Calvin cycle are sometimes called light-independent reactions. But don't let the name fool you... those reactions do require sunlight to work.
The protein RuBisCO also helps in the process to change carbon from the air into sugars. RuBisCO works slowly, so plants need a lot of it. In fact, RuBisCO is the most abundant protein in the world!
The products of the Calvin cycle are used to make the simple sugar glucose. Glucose is used to build more complex sugars like starch and cellulose. Starch stores energy for the plant and cellulose is the stuff of which plants are made.
XXX . ____ . 000 000 101010 22 How Energy Storage Works
The electricity grid is a complex system in which power supply and demand must be equal at any given moment. Constant adjustments to the supply are needed for predictable changes in demand, such as the daily patterns of human activity, as well as unexpected changes from equipment overloads and storms. Energy storage plays an important role in this balancing act and helps to create a more flexible and reliable grid system.

Energy storage technologies can help smooth out the electricity supply from variable energy sources like wind and solar power. Photo: germanborillo/Flickr
For example, when there is more supply than demand, such as during the night when low-cost power plants continue to operate, the excess electricity generation can be used to power storage devices. When demand is greater than supply, storage facilities can discharge their stored energy to the grid.
Pumping water back behind hydroelectric dams has been used for decades as a form of storage that absorbs excess capacity from the grid and returns capacity to the grid later when it is needed. In the future, as more storage technology options emerge and the United States transitions to a cleaner energy economy, energy storage is poised to play an even greater role.
The benefits of energy storage
Because some renewable energy technologies – such as wind and solar – have variable outputs, storage technologies have great potential for smoothing out the electricity supply from these sources and ensuring that the supply of generation matches the demand.

Energy storage can play an important role in creating a more flexible and reliable electricity system. Photo: Dennis Forgione/Flickr
Energy storage is also valued for its rapid response – most storage technologies can begin discharging power to the grid very quickly, while fossil fuel sources tend to take longer to ramp up. This rapid response is important for ensuring stability of the grid when unexpected increases in demand occur.
Energy storage also becomes more important the farther you are from the electrical grid. For example, when you turn on the lights in your home, the power comes from the grid; but when you turn on a flashlight while camping, you must rely on the stored energy in the batteries. Similarly, homes that are farther away from the transmission grid are more vulnerable to disruption than homes in large metropolitan areas. Islands and microgrids that are disconnected from the larger electrical grid system depend on energy storage to ensure power stability, just like you depend on the batteries in your flashlight while camping.
Current U.S. energy storage capacity
The U.S. has about 23 gigawatts (GW) of storage capacity, approximately equal to the capacity of 38 typical coal plants [1].
Pumped hydroelectric storage accounts for about 96 percent of this total storage capacity [2], most of which was built in the 1960s and 1970s to accompany the new fleet of nuclear power plants. Because nuclear power plants are not designed to ramp up or down, their generation is constant at all times of the day. When demand for electricity is low at night, pumped hydro facilities store the energy from nuclear plants for later use during peak demand. These pumped hydro plants have proven valuable for quickly adjusting to small changes in demand or supply.

Seneca Pumped Hydro Generating Station in northwest Pennsylvania. Water is pumped to the reservoir in the upper left during periods of low electricity demand, then released to generate electricity during periods of higher demand. Photo: U.S. Army Corps of Engineers
Emerging storage facilities will allow us to store energy generated from wind and solar resources on shorter time frames to smooth variability, and on longer cycles to replace ever more fossil fuel. By charging storage facilities with energy generated from renewable sources, we can reduce our greenhouse gas emissions and our dependence on fossil fuels.
While the U.S. electric grid does not necessarily need more storage now, storage capacity will become more important as wind, solar, and other variable renewable energy resources expand in the power mix. Studies have shown that the existing grid can accommodate a sizeable increase in variable generation [3], but there are many exciting technologies in development that could help us store energy in the future and support an even greater amount of renewable energy on the grid.
Energy storage technologies
Different energy storage technologies contribute to electricity stability by working at various stages of the grid, from generation to consumer end-use.
Thermal Storage
Thermal storage is used for electricity generation by using power from the sun, even when the sun is not shining. Concentrating solar plants can capture heat from the sun and store the energy in water, molten salts, or other fluids. This stored energy is later used to generate electricity, enabling the use of solar energy even after sunset.
Plants like these are currently operating or proposed in California, Arizona, and Nevada [4]. For example, the proposed Rice Solar Energy Project in Blythe, California will use a molten salt storage system with a concentrating solar tower to provide power for approximately 68,000 homes each year [5].

Concentrating solar plants focus the sun's heat to store energy in water, molten salts, or other fluids, which can be utilized even after the sun has set. Photo: NASA
Thermal storage technologies also exist for end-use energy storage. One method is freezing water at night using off-peak electricity, then releasing the stored cold energy from the ice to help with air conditioning during the day [6].
For example, Ice Energy’s Ice Bear system creates a block of ice at night, and then uses the ice during the day to condense the air conditioning system’s refrigerant [7]. In this way, the Ice Bear system shifts the building’s electricity consumption from the daytime peak to off-peak times when the electricity is less expensive. Additionally, the Bonneville Power Administration is conducting a pilot program on storing excess wind generation in residential water heaters [8].
Compressed Air
Compressed Air Energy Storage (CAES) also works as a generation storage technology by using the elastic potential energy of compressed air to improve the efficiencies of conventional gas turbines.
CAES systems compress air using electricity during off-peak times, and then store the air in underground caverns. During times of peak demand, the air is drawn from storage and fired with natural gas in a combustion turbine to generate electricity [9]. This method uses only a third of the natural gas used in conventional methods [10]. Because CAES plants require some sort of underground reservoir, they are limited by their locations. Two commercial CAES plants currently operate in Huntorf, Germany and MacIntosh, Alabama, though plants have been proposed in other parts of the United States.
Hydrogen
Hydrogen can be used as a zero-carbon fuel for generation. Excess electricity can be used to create hydrogen, which can be stored and used later in fuel cells, engines, or gas turbines to generate electricity without producing harmful emissions . NREL has studied the potential for creating hydrogen from wind power and storing it in the wind turbine towers for electricity generation when the wind isn’t blowing .

The Wind to Hydrogen Project at NREL studies the storage of wind energy as hydrogen. Photo: NREL
Pumped Hydroelectric Storage
Pumped hydroelectric storage offers a way to store energy at the grid’s transmission stage, by storing excess generation for later use.
Many hydroelectric power plants include two reservoirs at different elevations. These plants store energy by pumping water into the upper reservoir when supply exceeds demand. When demand exceeds supply, the water is released into the lower reservoir by running downhill through turbines to generate electricity.
With more than 22 GW of installed capacity in the United States, pumped hydro storage is the largest storage system operating today . However, the long permitting process and high cost of pumped storage makes further projects unlikely.
Flywheels
Flywheels can provide a variety of benefits to the grid at either the transmission or distributionlevel, by storing electricity in the form of a spinning mass.
The device is shaped liked a cylinder and contains a large rotor inside a vacuum. When the flywheel draws power from the grid, the rotor accelerates to very high speeds, storing the electricity as rotational energy. To discharge the stored energy, the rotor switches to generation mode, slows down, and runs on inertial energy, thus returning electricity to the grid .

A flywheel rotor, pictured here, is spun at high speed to store electricity as rotational energy. Photo: Wikimedia Commons
Flywheels typically have long lifetimes and require little maintenance. The devices also have high efficiencies and rapid response times. Because they can be placed almost anywhere, flywheels can be located close to the consumers and store electricity for distribution.
While a single flywheel device has a typical capacity on the order of kilowatts, many flywheels can be connected in a “flywheel farm” to create a storage facility on the order of megawatts . Beacon Power’s Stephentown Flywheel Energy Storage Plant in New York is the largest flywheel facility in the United States, with an operating capacity of 20 MW .
Batteries
Batteries, like those in a flashlight or cell phone, can also be used to store energy on a large scale.
Like flywheels, batteries can be located anywhere so they are often seen as storage for distribution, when a battery facility is located near consumers to provide power stability; or end-use, like batteries in electric vehicles.

Batteries can be located in communities to provide power stability for homes. Photo: Green Energy Futures/Flickr
There are many different types of batteries that have large-scale energy storage potential, including sodium-sulfur, metal air, lithium ion, and lead-acid batteries. There are several battery installations at wind farms; including the Notrees Wind Storage Demonstration Project in Texas, which uses a 36 MW battery facility to help ensure stability of the power supply even when the wind isn’t blowing [17].
Advancements in battery technologies have been made largely due to the expanding electric vehicle (EV) industry. As more developments are made with EVs, battery cost should continue to decline [18]. Electric vehicles could also have an impact on energy storage through vehicle-to-grid technologies, in which their batteries can be connected to the grid and discharge power for others to use.
The future of energy storage
As new energy storage technologies are researched and tested, some barriers are likely to slow the commercialization of these technologies.
Energy storage is expensive, especially without policies that place a monetary value on the unique benefits of storage. Plus there is no current need for additional storage capacity to maintain electricity grid reliability. Without an operational need, it is difficult for storage to be cost-effective in the present . Furthermore, storage lacks a robust track record of large commercial-scale projects (with the exception of pumped hydro), making it difficult to deploy new projects.
Despite these potential barriers, certain programs and policies can help drive the development and deployment of storage technologies. The Department of Energy’s Energy Storage Program researches different storage technologies and works closely with industry on pilot storage programs .

Research programs can help advance the deployment and commercialization of energy storage. Photo: Argonne National Laboratory/Flickr
The deployment of storage technologies can also be advanced through renewable electricity standards (RES). Some states recognize storage technologies as acceptable renewable generation in their RES, and other states award Renewable Energy Credits (REC) to energy generation from storage devices that were charged by renewables [21].
The Federal Energy Regulatory Commission (FERC), the agency that regulates the electricity grid, has created a pricing structure that pays storage technologies and other fast-ramping resources a higher price for their services. This pricing structure, called Pay-for-Performance, recognizes the value of rapid response in providing stability to the grid. Pay-for-Performance has the potential to make storage technologies more cost-effective on a commercial-scale. An investment tax credit (ITC) would also help accelerate the deployment of storage technologies.
With the support of government and industry, energy storage technologies can continue to develop and expand, aid in the increasing deployment of variable renewable energy sources, and help store an ever-growing amount of clean, renewable energy in the future.
++++++++++++++++++++++++++++++++++++++++++++++++++++++++++++++++++++++++++++++
GO REDEEMED TRANSPORT ENERGY ELECTRON AS LIKE AS BEE AND NUCLEAR SOURCES
HYPER PHYSICS LOOPING ENERGY SAVING
++++++++++++++++++++++++++++++++++++++++++++++++++++++++++++++++++++++++++++++
Tidak ada komentar:
Posting Komentar