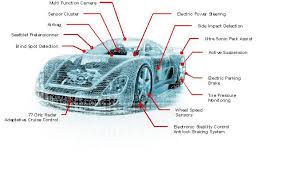

The theoretical basis of blocking is the following mathematical result. Given random variables, X and Y
\operatorname{Var}(X-Y)= \operatorname{Var}(X) + \operatorname{Var}(Y) - 2\operatorname{Cov}(X,Y).
The difference between the treatment and the control can thus be given minimum variance (i.e. maximum precision) by maximising the covariance (or the correlation) between X and Y.
This reduces sources of variability and thus leads to greater precision.
Use
Reducing known variability is exactly what blocking does. Its principle lies in the fact that a variability that cannot be overcome (e.g. needing two batches of raw material to produce 1 container of a chemical) is confounded or aliased with a(n) (higher/highest order) interaction to eliminate its influence on the end product. High order interactions are usually of the least importance (think of the fact that temperature of a reactor or the batch of raw materials is more important than the combination of the two - this is especially true when more (3, 4, ...) factors are present) thus it is preferable to confound this variability with the higher interaction.
Suppose a process is invented that intends to make the soles of shoes last longer, and a plan is formed to conduct a field trial. Given a group of n volunteers, one possible design would be to give n/2 of them shoes with the new soles and n/2 of them shoes with the ordinary soles, randomizing the assignment of the two kinds of soles. This type of experiment is a completely randomized design. Both groups are then asked to use their shoes for a period of time, and then measure the degree of wear of the soles. This is a workable experimental design, but purely from the point of view of statistical accuracy (ignoring any other factors), a better design would be to give each person one regular sole and one new sole, randomly assigning the two types to the left and right shoe of each volunteer. Such a design is called a randomized complete block design. This design will be more sensitive than the first, because each person is acting as their own control and thus the control group is more closely matched to the treatment group.
Theoretical basis
blocking instance variable
The
design of experiments (
DOE,
DOX, or
experimental design)
is the design of any task that aims to describe or explain the
variation of information under conditions that are hypothesized to
reflect the variation. The term is generally associated with true
experiments in which the design introduces conditions that directly affect the variation, but may also refer to the design of
quasi-experiments, in which
natural conditions that influence the variation are selected for observation.
In its simplest form, an experiment aims at predicting the outcome by
introducing a change of the preconditions, which is reflected in a
variable called the
predictor. The change in the predictor is generally hypothesized to result in a change in the second variable, hence called the
outcome
variable. Experimental design involves not only the selection of
suitable predictors and outcomes, but planning the delivery of the
experiment under statistically optimal conditions given the constraints
of available resources.
Main concerns in experimental design include the establishment of
validity, reliability, and
replicability.
For example, these concerns can be partially addressed by carefully
choosing the predictor, reducing the risk of measurement error, and
ensuring that the documentation of the method is sufficiently detailed.
Related concerns include achieving appropriate levels of
statistical power and
sensitivity.
Correctly designed experiments advance knowledge in the natural and
social sciences and engineering. Other applications include marketing
and policy making.
Lind limited his subjects to men who "were as similar as I could
have them", that is he provided strict entry requirements to reduce
extraneous variation. He divided them into six pairs, giving each pair
different supplements to their basic diet for two weeks. The treatments
were all remedies that had been proposed:
- A quart of cider every day.
- Twenty five gutts (drops) of vitriol (sulphuric acid) three times a day upon an empty stomach.
- One half-pint of seawater every day.
- A mixture of garlic, mustard, and horseradish in a lump the size of a nutmeg.
- Two spoonfuls of vinegar three times a day.
- Two oranges and one lemon every day.
The citrus treatment stopped after six days when they ran out of
fruit, but by that time one sailor was fit for duty while the other had
almost recovered. Apart from that, only group one (cider) showed some
effect of its treatment. The remainder of the crew presumably served as a
control, but Lind did not report results from any control (untreated)
group
A methodology for designing experiments was proposed by
Ronald Fisher, in his innovative books:
The Arrangement of Field Experiments (1926) and
The Design of Experiments
(1935). Much of his pioneering work dealt with agricultural
applications of statistical methods. As a mundane example, he described
how to test the
lady tasting tea hypothesis,
that a certain lady could distinguish by flavour alone whether the milk
or the tea was first placed in the cup. These methods have been broadly
adapted in the physical and social sciences, are still used in
agricultural engineering and differ from the design and analysis of
computer experiments.
- Comparison
- In some fields of study it is not possible to have independent measurements to a traceable metrology standard. Comparisons between treatments are much more valuable and are usually preferable, and often compared against a scientific control or traditional treatment that acts as baseline.
- Randomization
- Random assignment is the process of assigning individuals at random
to groups or to different groups in an experiment, so that each
individual of the population has the same chance of becoming a
participant in the study. The random assignment of individuals to groups
(or conditions within a group) distinguishes a rigorous, "true"
experiment from an observational study or "quasi-experiment".[12]
There is an extensive body of mathematical theory that explores the
consequences of making the allocation of units to treatments by means of
some random mechanism such as tables of random numbers, or the use of
randomization devices such as playing cards or dice. Assigning units to
treatments at random tends to mitigate confounding,
which makes effects due to factors other than the treatment to appear
to result from the treatment. The risks associated with random
allocation (such as having a serious imbalance in a key characteristic
between a treatment group and a control group) are calculable and hence
can be managed down to an acceptable level by using enough experimental
units. However, if the population is divided into several subpopulations
that somehow differ, and the research requires each subpopulation to be
equal in size, stratified sampling can be used. In that way, the units
in each subpopulation are randomized, but not the whole sample. The
results of an experiment can be generalized reliably from the
experimental units to a larger statistical population of units only if the experimental units are a random sample from the larger population; the probable error of such an extrapolation depends on the sample size, among other things.
- Statistical replication
- Measurements are usually subject to variation and measurement uncertainty;
thus they are repeated and full experiments are replicated to help
identify the sources of variation, to better estimate the true effects
of treatments, to further strengthen the experiment's reliability and
validity, and to add to the existing knowledge of the topic.[13]
However, certain conditions must be met before the replication of the
experiment is commenced: the original research question has been
published in a peer-reviewed
journal or widely cited, the researcher is independent of the original
experiment, the researcher must first try to replicate the original
findings using the original data, and the write-up should state that the
study conducted is a replication study that tried to follow the
original study as strictly as possible.[14]
- Blocking
- Blocking is the arrangement of experimental units into groups
(blocks/lots) consisting of units that are similar to one another.
Blocking reduces known but irrelevant sources of variation between units
and thus allows greater precision in the estimation of the source of
variation under study.
- Orthogonality
Example of orthogonal factorial design
- Orthogonality concerns the forms of comparison (contrasts) that can
be legitimately and efficiently carried out. Contrasts can be
represented by vectors and sets of orthogonal contrasts are uncorrelated
and independently distributed if the data are normal. Because of this
independence, each orthogonal treatment provides different information
to the others. If there are T treatments and T – 1 orthogonal contrasts, all the information that can be captured from the experiment is obtainable from the set of contrasts.
- Factorial experiments
- Use of factorial experiments instead of the one-factor-at-a-time
method. These are efficient at evaluating the effects and possible interactions of several factors (independent variables). Analysis of experiment design is built on the foundation of the analysis of variance,
a collection of models that partition the observed variance into
components, according to what factors the experiment must estimate or
test.
- This example is attributed to Harold Hotelling.It conveys some of the flavor of those aspects of the subject that involve combinatorial designs.
Weights of eight objects are measured using a pan balance
and set of standard weights. Each weighing measures the weight
difference between objects in the left pan vs. any objects in the right
pan by adding calibrated weights to the lighter pan until the balance is
in equilibrium. Each measurement has a random error. The average error is zero; the standard deviations of the probability distribution of the errors is the same number σ on different weighings; and errors on different weighings are independent. Denote the true weights by

We consider two different experiments:
- Weigh each object in one pan, with the other pan empty. Let Xi be the measured weight of the object, for i = 1, ..., 8.
- Do the eight weighings according to the following schedule and let Yi be the measured difference for i = 1, ..., 8:
-

- Then the estimated value of the weight θ1 is
-

- Similar estimates can be found for the weights of the other items. For example
-







The question of design of experiments is: which experiment is better?
The variance of the estimate
X1 of θ
1 is σ
2 if we use the first experiment. But if we use the second experiment, the variance of the estimate given above is σ
2/8.
Thus the second experiment gives us 8 times as much precision for the
estimate of a single item, and estimates all items simultaneously, with
the same precision. What the second experiment achieves with eight would
require 64 weighings if the items are weighed separately. However, note
that the estimates for the items obtained in the second experiment have
errors that correlate with each other.
Many problems of the design of experiments involve
combinatorial designs, as in this example and others.
Avoiding false positives
False positive conclusions, often resulting from the
pressure to publish or the author's own
confirmation bias,
are an inherent hazard in many fields. A good way to prevent biases
potentially leading to false positives in the data collection phase is
to use a double-blind design. When a double-blind design is used,
participants are randomly assigned to experimental groups but the
researcher is unaware of what participants belong to which group.
Therefore, the researcher can not affect the participants' response to
the intervention. Experimental designs with undisclosed degrees of
freedom are a problem. This can lead to conscious or unconscious "
p-hacking":
trying multiple things until you get the desired result. It typically
involves the manipulation - perhaps unconsciously - of the process of
statistical analysis and the degrees of freedom until they return a figure below the p<.05 level of statistical significance.
So the design of the experiment should include a clear statement
proposing the analyses to be undertaken. P-hacking can be prevented by
preregistering researches, in which researchers have to send their data
analysis plan to the journal they wish to publish their paper in before
they even start their data collection, so no data mining is possible .
Another way to prevent this is taking the double-blind design to the
data-analysis phase, where the data are sent to a data-analyst unrelated
to the research who scrambles up the data so there is no way to know
which participants belong to before they are potentially taken away as
outliers.
Clear and complete documentation of the experimental methodology is also important in order to support replication of results.
Discussion topics when setting up an experimental design
An experimental design or randomized clinical trial requires careful
consideration of several factors before actually doing the experiment.
An experimental design is the laying out of a detailed experimental
plan in advance of doing the experiment. Some of the following topics
have already been discussed in the principles of experimental design
section:
- How many factors does the design have? and are the levels of these factors fixed or random?
- Are control conditions needed, and what should they be?
- Manipulation checks; did the manipulation really work?
- What are the background variables?
- What is the sample size. How many units must be collected for the experiment to be generalisable and have enough power?
- What is the relevance of interactions between factors?
- What is the influence of delayed effects of substantive factors on outcomes?
- How do response shifts affect self-report measures?
- How feasible is repeated administration of the same measurement
instruments to the same units at different occasions, with a post-test
and follow-up tests?
- What about using a proxy pretest?
- Are there lurking variables?
- Should the client/patient, researcher or even the analyst of the data be blind to conditions?
- What is the feasibility of subsequent application of different conditions to the same units?
- How many of each control and noise factors should be taken into account?
The independent variable of a study often has many levels or
different groups. In a true experiment, researchers can have an
experimental group, which is where their intervention testing the
hypothesis is implemented, and a control group, which has all the same
element as the experimental group, without the interventional element.
Thus, when everything else except for one intervention is held constant,
researchers can certify with some certainty that this one element is
what caused the observed change. In some instances, having a control
group is not ethical. This is sometimes solved using two different
experimental groups. In some cases, independent variables are not
manipulable, for example when testing the difference between two groups
who have a different disease, or testing the difference between genders
(obviously variables that would be hard or unethical to assign
participants to). In these cases, a quasi-experimental design may be
used.
Causal attributions
In the pure experimental design, the independent (predictor) variable
is manipulated by the researcher - that is - every participant of the
research is chosen randomly from the population, and each participant
chosen is assigned randomly to conditions of the independent variable.
Only when this is done is it possible to certify with high probability
that the reason for the differences in the outcome variables are caused
by the different conditions. Therefore, researchers should choose the
experimental design over other design types whenever possible. However,
the nature of the independent variable does not always allow for
manipulation. In those cases, researchers must be aware of not
certifying about causal attribution when their design doesn't allow for
it. For example, in observational designs, participants are not assigned
randomly to conditions, and so if there are differences found in
outcome variables between conditions, it is likely that there is
something other than the differences between the conditions that causes
the differences in outcomes, that is - a third variable. The same goes
for studies with correlational design. (Adér & Mellenbergh, 2008).
Statistical control
It is best that a process be in reasonable statistical control prior
to conducting designed experiments. When this is not possible, proper
blocking, replication, and randomization allow for the careful conduct
of designed experiments. To control for nuisance variables, researchers institute
control checks
as additional measures. Investigators should ensure that uncontrolled
influences (e.g., source credibility perception) do not skew the
findings of the study. A
manipulation check
is one example of a control check. Manipulation checks allow
investigators to isolate the chief variables to strengthen support that
these variables are operating as planned.
One of the most important requirements of experimental research designs is the necessity of eliminating the effects of
spurious, intervening, and
antecedent variables.
In the most basic model, cause (X) leads to effect (Y). But there could
be a third variable (Z) that influences (Y), and X might not be the
true cause at all. Z is said to be a spurious variable and must be
controlled for. The same is true for
intervening variables
(a variable in between the supposed cause (X) and the effect (Y)), and
anteceding variables (a variable prior to the supposed cause (X) that is
the true cause). When a third variable is involved and has not been
controlled for, the relation is said to be a
zero order[disambiguation needed]
relationship. In most practical applications of experimental research
designs there are several causes (X1, X2, X3). In most designs, only one
of these causes is manipulated at a time.
Experimental designs after Fisher
Some efficient designs for estimating several main effects were found independently and in near succession by
Raj Chandra Bose and K. Kishen in 1940 at the
Indian Statistical Institute, but remained little known until the
Plackett-Burman designs were published in
Biometrika in 1946. About the same time,
C. R. Rao introduced the concepts of
orthogonal arrays as experimental designs. This concept played a central role in the development of
Taguchi methods by
Genichi Taguchi,
which took place during his visit to Indian Statistical Institute in
early 1950s. His methods were successfully applied and adopted by
Japanese and Indian industries and subsequently were also embraced by US
industry albeit with some reservations.
In 1950,
Gertrude Mary Cox and
William Gemmell Cochran published the book
Experimental Designs, which became the major reference work on the design of experiments for statisticians for years afterwards.
Developments of the theory of
linear models have encompassed and surpassed the cases that concerned early writers. Today, the theory rests on advanced topics in
linear algebra,
algebra and
combinatorics.
As with other branches of statistics, experimental design is pursued using both
frequentist and
Bayesian approaches: In evaluating statistical procedures like experimental designs,
frequentist statistics studies the
sampling distribution while
Bayesian statistics updates a
probability distribution on the parameter space.
Human participant experimental design constraints
Laws and ethical considerations preclude some carefully designed
experiments with human subjects. Legal constraints are dependent on
jurisdiction. Constraints may involve
institutional review boards,
informed consent and
confidentiality affecting both clinical (medical) trials and behavioral and social science experiments. In the field of toxicology, for example, experimentation is performed on laboratory
animals with the goal of defining safe exposure limits for
humans.Balancing the constraints are views from the medical field.
Regarding the randomization of patients, "... if no one knows which
therapy is better, there is no ethical imperative to use one therapy or
another." (p 380) Regarding experimental design, "...it is clearly not
ethical to place subjects at risk to collect data in a poorly designed
study when this situation can be easily avoided...". (p 393)
Plackett–Burman designs are
experimental designs presented in 1946 by
Robin L. Plackett and
J. P. Burman while working in the British
Ministry of Supply. Their goal was to find experimental designs for investigating the dependence of some measured quantity on a number of
independent variables (factors), each taking
L levels, in such a way as to minimize the
variance
of the estimates of these dependencies using a limited number of
experiments. Interactions between the factors were considered
negligible. The solution to this problem is to find an experimental
design where
each combination of levels for any
pair of factors appears the
same number of times, throughout all the experimental runs (refer table). A complete
factorial design would satisfy this criterion, but the idea was to find smaller designs.
Plackett–Burman design for 12 runs and 11 two-level factors[2] For any two Xi, each combination ( --, -+, +-, ++) appears three - i.e. the same number of times.
Run |
X1 |
X2 |
X3 |
X4 |
X5 |
X6 |
X7 |
X8 |
X9 |
X10 |
X11 |
1 |
+ |
+ |
+ |
+ |
+ |
+ |
+ |
+ |
+ |
+ |
+ |
2 |
− |
+ |
− |
+ |
+ |
+ |
− |
− |
− |
+ |
− |
3 |
− |
− |
+ |
− |
+ |
+ |
+ |
− |
− |
− |
+ |
4 |
+ |
− |
− |
+ |
− |
+ |
+ |
+ |
− |
− |
− |
5 |
− |
+ |
− |
− |
+ |
− |
+ |
+ |
+ |
− |
− |
6 |
− |
− |
+ |
− |
− |
+ |
− |
+ |
+ |
+ |
− |
7 |
− |
− |
− |
+ |
− |
− |
+ |
− |
+ |
+ |
+ |
8 |
+ |
− |
− |
− |
+ |
− |
− |
+ |
− |
+ |
+ |
9 |
+ |
+ |
− |
− |
− |
+ |
− |
− |
+ |
− |
+ |
10 |
+ |
+ |
+ |
− |
− |
− |
+ |
− |
− |
+ |
− |
11 |
− |
+ |
+ |
+ |
− |
− |
− |
+ |
− |
− |
+ |
12 |
+ |
− |
+ |
+ |
+ |
− |
− |
− |
+ |
− |
− |
For the case of two levels (
L=2), Plackett and Burman used the
method found in 1933 by
Raymond Paley for generating
orthogonal matrices whose elements are all either 1 or -1 (
Hadamard matrices). Paley's method could be used to find such matrices of size
N for most
N equal to a multiple of 4. In particular, it worked for all such
N up to 100 except
N = 92. If
N is a power of 2, however, the resulting design is identical to a
fractional factorial design, so Plackett–Burman designs are mostly used when
N is a multiple of 4 but not a power of 2 (i.e.
N = 12, 20, 24, 28, 36 …). If one is trying to estimate less than
N parameters (including the overall average), then one simply uses a subset of the columns of the matrix.
For the case of more than two levels, Plackett and Burman rediscovered designs that had previously been given by
Raj Chandra Bose and
K. Kishen at the
Indian Statistical Institute.
[4] Plackett and Burman give specifics for designs having a number of experiments equal to the number of levels
L to some integer power, for
L = 3, 4, 5, or 7.
When interactions between factors are not negligible, they are often
confounded in Plackett–Burman designs with the main effects, meaning
that the designs do not permit one to distinguish between certain main
effects and certain interactions. This is called
aliasing or
confounding.
Extended uses
In 1993, Dennis Lin described a construction method via
half-fractions of Plackett-Burman designs, using one column to take half
of the rest of the columns. The resulting matrix, minus that column, is a "supersaturated design" for finding significant first order effects, under the assumption that few exist.
Box-Behnken designs can be made smaller, or very large ones constructed, by replacing the
fractional factorials and
incomplete blocks
traditionally used for plan and seed matrices, respectively, with
Plackett-Burmans. For example, a quadratic design for 30 variables
requires a 30 column PB plan matrix of zeroes and ones, replacing the
ones in each line using PB seed matrices of -1s and +1s (for 15 or 16
variables) wherever a one appears in the plan matrix, creating a 557
runs design with values, -1, 0, +1, to estimate the 496 parameters of a
full quadratic model.
By equivocating certain columns with parameters to be estimated,
Plackett-Burmans can also be used to construct mixed categorical and
numerical designs, with interactions or high order effects, requiring no
more than 4 runs more than the number of model parameters to be
estimated. Sort on columns assigned to categorical variable "A", defined
as A = 1+int(
a*
i /(max(
i)+.00001)) where
i is row number and
a
is A's number of values. Next sort on columns assigned to any other
categorical variables and repeat as needed. Such designs, if large, may
otherwise be incomputable by standard search techniques like
D-Optimality.
For example, 13 variables averaging 3 values each could have well over a
million combinations to search. To estimate roughly 100 parameters for a
nonlinear model in 13 variables must formally exclude from
consideration or compute |X'X| for well over 10
6C10
2 or roughly 10
600 matrices.
Computer simulations
are constructed to emulate a physical system. Because these are meant
to replicate some aspect of a system in detail, they often do not yield
an analytic solution. Therefore, methods such as
discrete event simulation or
finite element solvers are used. A
computer model is used to make inferences about the system it replicates. For example,
climate models are often used because experimentation on an earth sized object is impossible.
Objectives
Computer experiments have been employed with many purposes in mind. Some of those include:
- Uncertainty quantification:
Characterize the uncertainty present in a computer simulation arising
from unknowns during the computer simulation's construction.
- Inverse problems: Discover the underlying properties of the system from the physical data.
- Bias correction: Use physical data to correct for bias in the simulation.
- Data assimilation: Combine multiple simulations and physical data sources into a complete predictive model.
- Systems design: Find inputs that result in optimal system performance measures.
Computer simulation modeling

Modeling of computer experiments typically uses a Bayesian framework.
Bayesian statistics is an interpretation of the field of
statistics where which all evidence about the true state of the world is explicitly expressed in the form of
probabilities. In the realm of computer experiments, the Bayesian interpretation would imply we must form a
prior distribution
that represents our prior belief on the structure of the computer
model. The use of this philosophy for computer experiments started in
the 1980s and is nicely summarized by Sacks et al. (1989) . While the Bayesian approach is widely used,
frequentist approaches have been recently discussed .
The basic idea of this framework is to model the computer simulation
as an unknown function of a set of inputs. The computer simulation is
implemented as a piece of computer code that can be evaluated to produce
a collection of outputs. Examples of inputs to these simulations are
coefficients in the underlying model,
initial conditions and
forcing functions. It is natural to see the simulation as a deterministic function that maps these
inputs into a collection of
outputs. On the basis of seeing our simulator this way, it is common to refer to the collection of inputs as

, the computer simulation itself as

, and the resulting output as

. Both

and

are vector quantities, and they can be very large collections of
values, often indexed by space, or by time, or by both space and time.
Although

is known in principle, in practice this is not the case. Many
simulators comprise tens of thousands of lines of high-level computer
code, which is not accessible to intuition. For some simulations, such
as climate models, evaluation of the output for a single set of inputs
can require millions of computer hours .
Gaussian process prior
The typical model for a computer code output is a Gaussian process. For notational simplicity, assume

is a scalar. Owing to the Bayesian framework, we fix our belief that the function

follows a
Gaussian process,

where

is the mean function and

is the covariance function. Popular mean functions are low order polynomials and a popular
covariance function is
Matern covariance, which includes both the exponential (

) and Gaussian covariances (as

).
Design of computer experiments
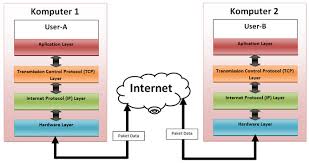
The design of computer experiments has considerable differences from
design of experiments
for parametric models. Since a Gaussian process prior has an infinite
dimensional representation, the concepts of A and D criteria (see
Optimal design),
which focus on reducing the error in the parameters, cannot be used.
Replications would also be wasteful in cases when the computer
simulation has no error. Criteria that are used to determine a good
experimental design include integrated mean squared prediction error and distance based criteria .
Popular strategies for design include
latin hypercube sampling and
low discrepancy sequences.
Problems with massive sample sizes
Unlike physical experiments, it is common for computer experiments to
have thousands of different input combinations. Because the standard
inference requires
matrix inversion of a square matrix of the size of the number of samples (

), the cost grows on the

.
Matrix inversion of large, dense matrices can also cause induce
numerical inaccuracies. Currently, this problem is solved by greedy
decision tree techniques, allowing effective computations for unlimited
dimensionality and sample size
patent WO2013055257A1, or avoided by using approximation methods, e.g. .
The
instrument effect is an issue in
experimental methodology meaning that any change during the measurement, or, the instrument, may influence the research validity. For example, in a
control group
design experiment, if the instruments used to measure the performance
of the experiment group and the control group are different, a wrong
conclusion about the experiment would be reached, the research result
would be invalid
Loss functions in statistical theory
Traditionally, statistical methods have relied on
mean-unbiased estimators of
treatment effects: Under the conditions of the
Gauss-Markov theorem,
least squares
estimators have minimum variance among all mean-unbiased estimators.
The emphasis on comparisons of means also draws (limiting) comfort from
the
law of large numbers, according to which the
sample means converge to the true mean. Fisher's textbook on the
design of experiments emphasized comparisons of treatment means.
However, loss functions were avoided by
Ronald A. Fisher.
[6]